Gesture recognition method based on variational mode decomposition and support vector machine
A variational modal decomposition and support vector machine technology, applied in the field of surface EMG signal recognition, to achieve the effect of less deep learning, solving modal aliasing, and improving the efficiency of finding parameters
- Summary
- Abstract
- Description
- Claims
- Application Information
AI Technical Summary
Problems solved by technology
Method used
Image
Examples
Embodiment Construction
[0100] The present invention will be further described below in conjunction with the accompanying drawings and specific embodiments.
[0101] Such as figure 1 As shown, the main steps of the gesture recognition method based on variational mode decomposition and support vector machine are:
[0102] Step 1.1: collect surface electromyographic signals, construct training set and test set;
[0103] Step 1.2: Randomly extract a surface EMG signal from the constructed SEMG training set for variational mode decomposition to obtain the variational modal function components u k , the modal component u k The nth update formula is:
[0104]
[0105] where α is the penalty factor, is the Fourier transform of the surface EMG signal of the gesture, is the Fourier transform of the Lagrange multipliers, is the Fourier transform of the ith modal component, is the nth update of the K modal component, n is the number of updates of the expression (1), ranging from 0 to K-1, ω is the...
PUM
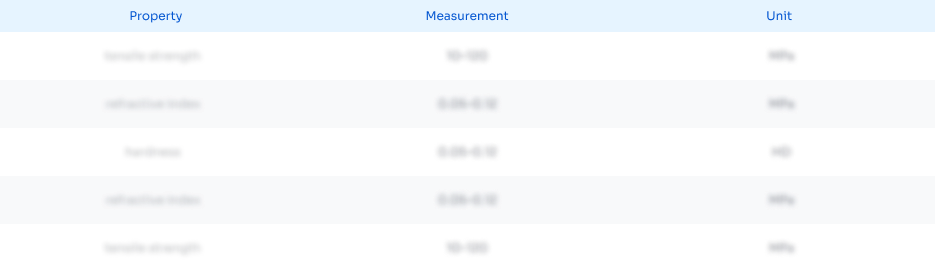
Abstract
Description
Claims
Application Information
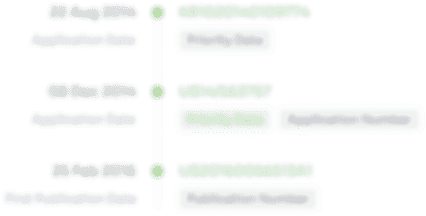
- R&D Engineer
- R&D Manager
- IP Professional
- Industry Leading Data Capabilities
- Powerful AI technology
- Patent DNA Extraction
Browse by: Latest US Patents, China's latest patents, Technical Efficacy Thesaurus, Application Domain, Technology Topic, Popular Technical Reports.
© 2024 PatSnap. All rights reserved.Legal|Privacy policy|Modern Slavery Act Transparency Statement|Sitemap|About US| Contact US: help@patsnap.com