Brain tumor detection method based on attention mechanism and MRI (Magnetic Resonance Imaging) multi-modal fusion
A detection method and attention technology, applied in the intersection of computer and medicine, in the field of deep learning, which can solve the problems of huge data volume, unclear outline, and large 3D convolution kernel.
- Summary
- Abstract
- Description
- Claims
- Application Information
AI Technical Summary
Problems solved by technology
Method used
Image
Examples
Embodiment Construction
[0056] The present invention will be described in detail below in conjunction with the accompanying drawings and embodiments.
[0057] A brain tumor detection method based on attention mechanism and MRI multimodal fusion, the method comprises the following steps,
[0058] Step S1: Dataset introduction.
[0059] In order to verify the performance of the model, Brats2015 is selected as the data set. The entire data set contains 274 samples, of which 220 samples are HGG cases, medically known as high-grade glioma, which is a poorly differentiated malignant tumor; the remaining 54 samples are LGG cases, medically known as low-grade glioma Tumors, these are benign tumors with good differentiation properties. Each sample in the dataset contains 5 3D Volumes, and each 3D Volume consists of 155 layers of 2D images. The first four 3D images are MRI scan results [T1, T2, T1c, Flair], which represent the basic structure, tissue water content, tissue blood supply, and tissue bound wate...
PUM
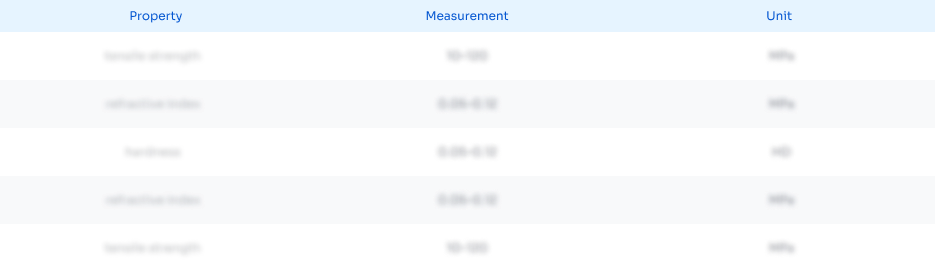
Abstract
Description
Claims
Application Information
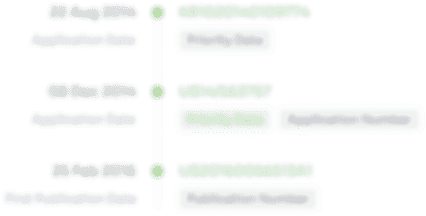
- R&D Engineer
- R&D Manager
- IP Professional
- Industry Leading Data Capabilities
- Powerful AI technology
- Patent DNA Extraction
Browse by: Latest US Patents, China's latest patents, Technical Efficacy Thesaurus, Application Domain, Technology Topic, Popular Technical Reports.
© 2024 PatSnap. All rights reserved.Legal|Privacy policy|Modern Slavery Act Transparency Statement|Sitemap|About US| Contact US: help@patsnap.com