Multi-stage unsupervised domain adaptive causal relationship identification method
A technology of causality and recognition methods, applied in neural learning methods, character and pattern recognition, special data processing applications, etc., can solve problems such as forgetting disasters, limiting model capabilities, fuzzy classification boundaries, etc., to avoid forgetting disasters and retain diversity Sexuality, damage reduction effect
- Summary
- Abstract
- Description
- Claims
- Application Information
AI Technical Summary
Problems solved by technology
Method used
Image
Examples
Embodiment Construction
[0043] Specific embodiments of the present invention will be further described below in conjunction with the accompanying drawings.
[0044] The present invention is used for causal relationship identification for unsupervised domain adaptation. figure 1 It is a flow chart of the unsupervised domain adaptive causality identification method of the present invention. see figure 1 , the unsupervised domain adaptive causality identification method provided by the present invention specifically includes:
[0045] Step 1: Partition the source domain dataset. Three sets of source domain datasets are obtained by randomly dividing the source domain dataset three times. Each division uses the probability of 60:20:20 to divide the original dataset into training set, test set and verification set. Repeat steps 2 and 3 below for these three sets of source domain datasets to obtain three sets of target domain models.
[0046]Step 2: Conduct pre-training based on contrastive learning. U...
PUM
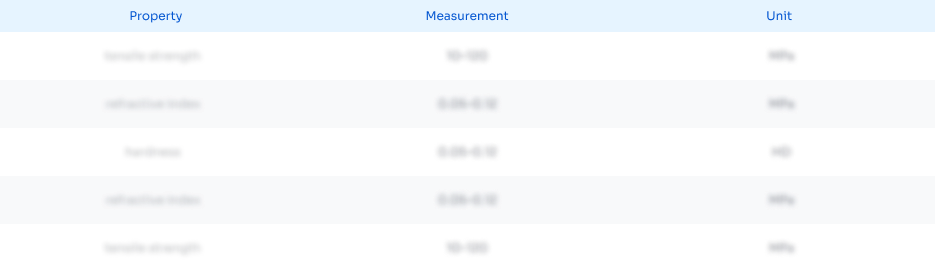
Abstract
Description
Claims
Application Information
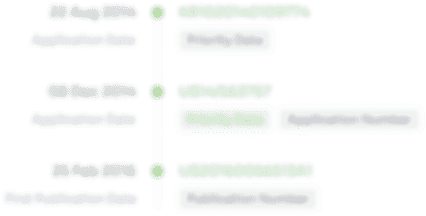
- R&D Engineer
- R&D Manager
- IP Professional
- Industry Leading Data Capabilities
- Powerful AI technology
- Patent DNA Extraction
Browse by: Latest US Patents, China's latest patents, Technical Efficacy Thesaurus, Application Domain, Technology Topic, Popular Technical Reports.
© 2024 PatSnap. All rights reserved.Legal|Privacy policy|Modern Slavery Act Transparency Statement|Sitemap|About US| Contact US: help@patsnap.com