Medical image automatic segmentation method based on deep learning
A technology for automatic segmentation of medical images, applied in the field of medical image processing, can solve problems such as difficult detection of small targets and blurred boundaries of segmented targets
- Summary
- Abstract
- Description
- Claims
- Application Information
AI Technical Summary
Problems solved by technology
Method used
Image
Examples
Embodiment 1
[0030] A method for automatic segmentation of medical images based on deep learning, the specific implementation steps are as follows:
[0031] (1) Obtain the patient's original medical image and the artificial segmentation results of the target area in the image from the medical image public data set, and construct a training data set;
[0032] (2) Build as figure 1 The U-shaped deep convolutional network that fuses the residual module and the attention mechanism shown is called RA-Unet, including:
[0033](2-a) U-shaped network is used as the backbone network. The backbone network consists of five encoding layers, four skip connection layers, four decoding layers and one 1×1 convolutional layer. The output of the first encoding layer Not only as the input of the second encoding layer, but also connected to the fourth decoding layer through the first skip connection layer as the input of the decoding layer; the output of the second encoding layer is not only used as the inpu...
Embodiment 2
[0048] The method in Example 1 was used to conduct experiments on the MICCAI 2017 pancreas public segmentation dataset. There are two segmentation categories: background, pancreas organ. The computer environment of this experiment: the operating system is Windows 10; an NVIDIA RTX308010G GPU; the software platform is: Python3 and PyTorch 1.6.
[0049] In this embodiment, the original CT images of 80 cases and the corresponding artificial segmentation results of the pancreas are selected from the pancreas public segmentation data set as the training data set, and the original CT images of 20 cases are selected as the test data set. In the experiment, the average Dice coefficient was used as the evaluation standard, and the segmentation method of the present invention achieved a Dice coefficient of 85.12% on the task of pancreas segmentation, and obtained a relatively high segmentation accuracy. The partial image segmentation results of the test set are as follows: Figure 4 (...
Embodiment 3
[0051] Using the method in Example 1, the kidney organ segmentation experiment was performed on the MICCAI 2017 kidney public segmentation dataset. There are two segmentation categories: background, kidney organ. The computer environment of this experiment: the operating system is Windows 10; an NVIDIA RTX3080 10G GPU; the software platform is: Python3 and PyTorch 1.6.
[0052] In this embodiment, the original CT images of 126 cases and the corresponding artificial kidney segmentation results are selected from the kidney public segmentation data set as the training data set, and the CT images of 84 cases are selected as the test data set. In the experiment, the average Dice coefficient was used as the evaluation standard, and the segmentation method of the present invention achieved a Dice coefficient of 91.12% on the pancreas segmentation task. The partial image segmentation results of the test set are as follows: Figure 5 (a)~ Figure 5 As shown in (d), the area shown by...
PUM
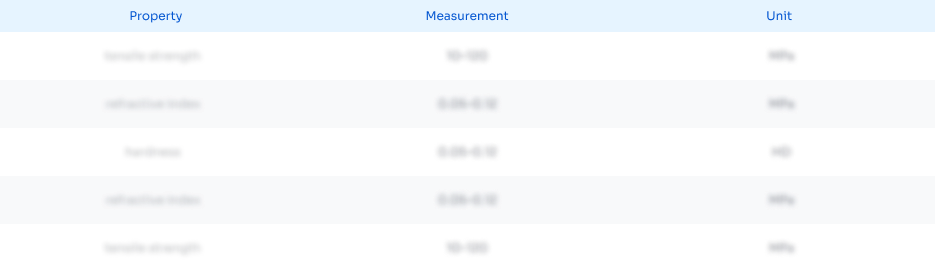
Abstract
Description
Claims
Application Information
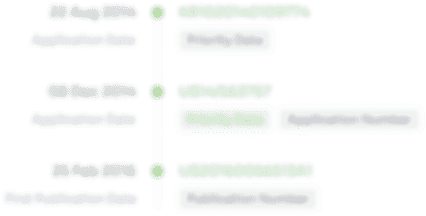
- R&D
- Intellectual Property
- Life Sciences
- Materials
- Tech Scout
- Unparalleled Data Quality
- Higher Quality Content
- 60% Fewer Hallucinations
Browse by: Latest US Patents, China's latest patents, Technical Efficacy Thesaurus, Application Domain, Technology Topic, Popular Technical Reports.
© 2025 PatSnap. All rights reserved.Legal|Privacy policy|Modern Slavery Act Transparency Statement|Sitemap|About US| Contact US: help@patsnap.com