Multi-wind-field wind speed space-time prediction method based on graph convolution and recurrent neural network
A technology of cyclic neural network and forecasting method, which is applied in the field of wind speed forecasting, can solve problems such as difficult data processing in non-European space, and achieve the effect of ensuring safe and stable economic operation
- Summary
- Abstract
- Description
- Claims
- Application Information
AI Technical Summary
Problems solved by technology
Method used
Image
Examples
Embodiment Construction
[0035] Below in conjunction with specific embodiment, further illustrate the present invention, should be understood that these embodiments are only used to illustrate the present invention and are not intended to limit the scope of the present invention, after having read the present invention, those skilled in the art will understand various equivalent forms of the present invention All modifications fall within the scope defined by the appended claims of the present application.
[0036] The present invention provides a multi-wind field wind speed spatio-temporal prediction method based on graph convolution and cyclic neural network, such as figure 1 As shown, the method includes the following steps:
[0037] Step (1): Obtain the location information and wind speed data of several wind farms in a certain area, use the undirected weighted graph to model the data, and use the spectral graph convolution on the established undirected weighted graph Calculate, realize the preli...
PUM
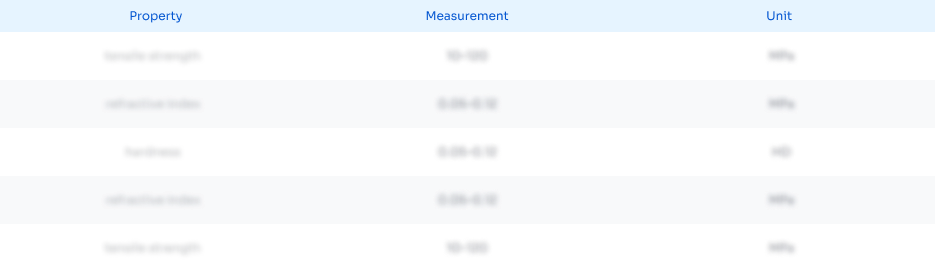
Abstract
Description
Claims
Application Information
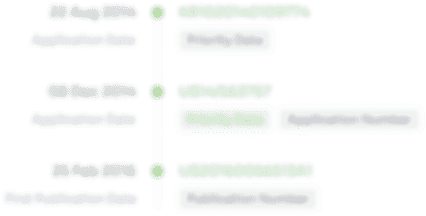
- R&D Engineer
- R&D Manager
- IP Professional
- Industry Leading Data Capabilities
- Powerful AI technology
- Patent DNA Extraction
Browse by: Latest US Patents, China's latest patents, Technical Efficacy Thesaurus, Application Domain, Technology Topic, Popular Technical Reports.
© 2024 PatSnap. All rights reserved.Legal|Privacy policy|Modern Slavery Act Transparency Statement|Sitemap|About US| Contact US: help@patsnap.com