Wind power prediction method based on self-learning radial basis function support vector machine
A wind power forecasting and support vector machine technology, which is applied in forecasting, information technology support systems, data processing applications, etc., can solve the problems of wind power generation uncertainty, uncontrollable power grid safe and stable economic operation, etc., to achieve safe and stable Economical operation, optimized grid scheduling, and high-precision effects
- Summary
- Abstract
- Description
- Claims
- Application Information
AI Technical Summary
Problems solved by technology
Method used
Image
Examples
Embodiment Construction
[0042] The preferred embodiments of the present invention will be described below in conjunction with the accompanying drawings. It should be understood that the preferred embodiments described here are only used to illustrate and explain the present invention, and are not intended to limit the present invention.
[0043] A wind power prediction method based on self-learning radial basis kernel function support vector machine, including:
[0044] Obtain the steps of obtaining the SVM model through model training;
[0045] And a step of inputting the data required for wind power prediction into the SVM model obtained from the above training to obtain a prediction result.
[0046] Wherein, the steps of obtaining the SVM model through model training specifically include:
[0047] Step 101, model training basic data input;
[0048] Step 102, preprocessing the above-mentioned input training basic data;
[0049] Step 103, SVM classifier training;
[0050] Step 104, by inputting ...
PUM
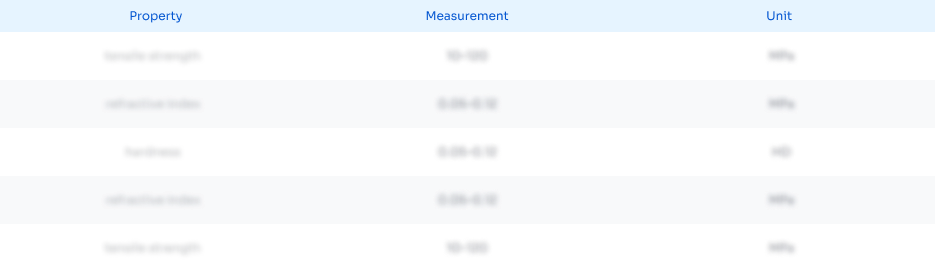
Abstract
Description
Claims
Application Information
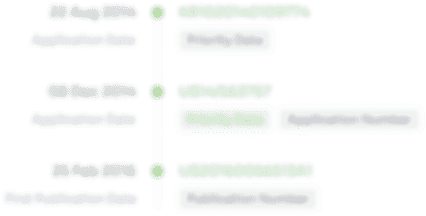
- R&D Engineer
- R&D Manager
- IP Professional
- Industry Leading Data Capabilities
- Powerful AI technology
- Patent DNA Extraction
Browse by: Latest US Patents, China's latest patents, Technical Efficacy Thesaurus, Application Domain, Technology Topic, Popular Technical Reports.
© 2024 PatSnap. All rights reserved.Legal|Privacy policy|Modern Slavery Act Transparency Statement|Sitemap|About US| Contact US: help@patsnap.com