Multi-factor electrical load prediction method based on deep learning
A technology of electric load and deep learning, which is applied to load forecasting, neural learning methods, electrical components, etc. in AC networks, and can solve the problem of large differences between forecasted results and real values, inability to consider meteorological data, and poor forecasting accuracy and other issues to achieve the effect of improving prediction accuracy, improving prediction accuracy, and reducing impact
- Summary
- Abstract
- Description
- Claims
- Application Information
AI Technical Summary
Problems solved by technology
Method used
Image
Examples
Embodiment Construction
[0067] The present invention will be described in further detail below in conjunction with the accompanying drawings and specific embodiments. The present invention introduces external environmental factors such as temperature, humidity, wind, etc., which are the most influential factors of electricity load, and uses k-nearest (KNN) algorithm and improved DBSCAN (Density-Based Spatial Clustering of Applications with Noise-based spatial clustering Application in noise) algorithm for abnormal data detection, autoregressive interpolation, and sequence data normalization to preprocess and standardize the data; then propose an improved CNN-LSTM electricity load forecasting model, first using the CNN feature extraction module Learn the local features of the input data; then input it into the LSTM sequence learning model to extract the sequence feature information of the input data; at the same time, introduce the self-attention mechanism into the LSTM to learn the features of the LST...
PUM
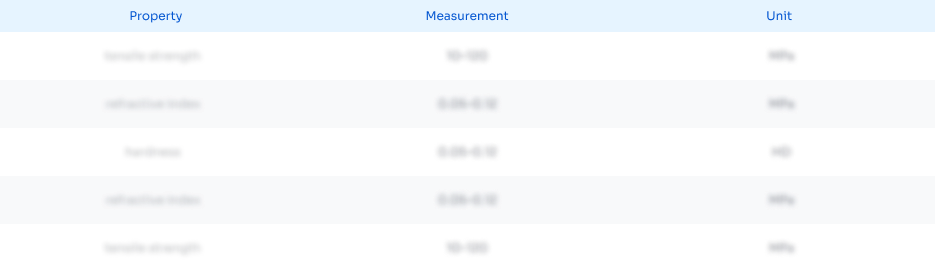
Abstract
Description
Claims
Application Information
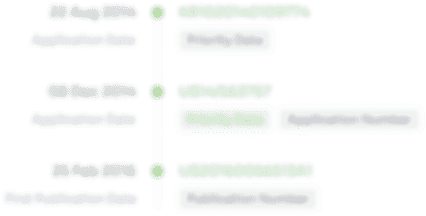
- R&D Engineer
- R&D Manager
- IP Professional
- Industry Leading Data Capabilities
- Powerful AI technology
- Patent DNA Extraction
Browse by: Latest US Patents, China's latest patents, Technical Efficacy Thesaurus, Application Domain, Technology Topic, Popular Technical Reports.
© 2024 PatSnap. All rights reserved.Legal|Privacy policy|Modern Slavery Act Transparency Statement|Sitemap|About US| Contact US: help@patsnap.com