Lithium battery nuclear temperature evaluation method and system based on transfer learning
A transfer learning and lithium battery technology, applied in neural learning methods, biological neural network models, design optimization/simulation, etc., can solve problems such as explosion, data not in the same distribution, gradient disappearance, etc., to improve training efficiency, The effect of speeding up training progress and reducing training time
- Summary
- Abstract
- Description
- Claims
- Application Information
AI Technical Summary
Problems solved by technology
Method used
Image
Examples
Embodiment 1
[0044] like figure 1 As shown, Embodiment 1 of the present invention provides a lithium battery nuclear temperature assessment method based on transfer learning, including the following process:
[0045] Obtain the lithium battery parameter data in the target domain;
[0046] According to the obtained lithium battery parameter data and the target domain neural network model, the evaluation result of the lithium battery core temperature is obtained;
[0047] Among them, the source domain neural network model is obtained according to the historical data training of the source domain lithium battery, and the fully connected layer of the source domain neural network model is retrained according to the target domain data to obtain the target domain neural network.
[0048] Specifically, including:
[0049] Step 1: Obtain lithium battery data through BMS, including current, voltage, and surface temperature, and drill holes in the negative electrode of the battery to embed sensors to...
Embodiment 2
[0086] Embodiment 2 of the present invention provides a lithium battery nuclear temperature evaluation system based on transfer learning, including:
[0087] The data acquisition module is configured to: acquire the lithium battery parameter data in the target domain;
[0088] The nuclear temperature evaluation module is configured to: obtain the lithium battery nuclear temperature evaluation result according to the obtained lithium battery parameter data and the target domain neural network model;
[0089] Among them, the source domain neural network model is obtained according to the historical data training of the source domain lithium battery, and the fully connected layer of the source domain neural network model is retrained according to the target domain data to obtain the target domain neural network.
[0090] The working method of the system is the same as the transfer learning-based lithium battery nuclear temperature assessment method provided in Embodiment 1, and w...
Embodiment 3
[0092] Embodiment 3 of the present invention provides a computer-readable storage medium on which a program is stored, and is characterized in that, when the program is executed by a processor, the lithium battery core temperature based on transfer learning as described in Embodiment 1 of the present invention is realized. Steps in the evaluation method.
PUM
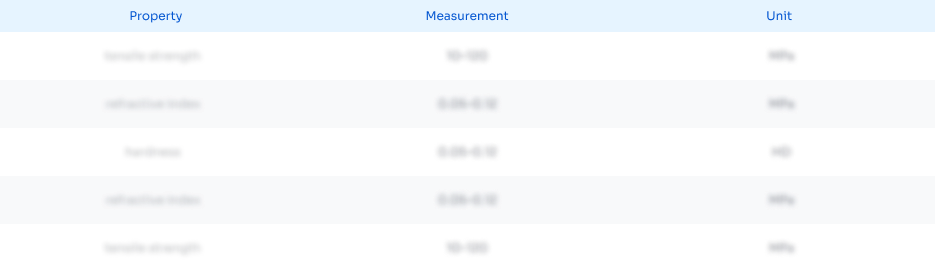
Abstract
Description
Claims
Application Information
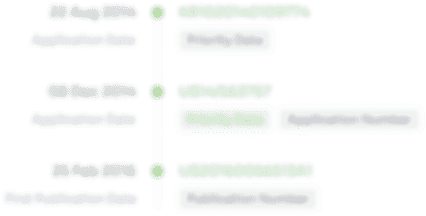
- R&D
- Intellectual Property
- Life Sciences
- Materials
- Tech Scout
- Unparalleled Data Quality
- Higher Quality Content
- 60% Fewer Hallucinations
Browse by: Latest US Patents, China's latest patents, Technical Efficacy Thesaurus, Application Domain, Technology Topic, Popular Technical Reports.
© 2025 PatSnap. All rights reserved.Legal|Privacy policy|Modern Slavery Act Transparency Statement|Sitemap|About US| Contact US: help@patsnap.com