Agent-driven multi-objective evolutionary fuzzy clustering method based on preference information
A fuzzy clustering method and multi-objective evolution technology, applied in the field of image processing, can solve the problems of low calculation efficiency and long time consumption of the algorithm, and achieve better segmentation effect, reduce time cost, and improve evolution efficiency
- Summary
- Abstract
- Description
- Claims
- Application Information
AI Technical Summary
Problems solved by technology
Method used
Image
Examples
Embodiment 1
[0058] Refer to attached figure 1 , an agent-driven multi-objective evolutionary fuzzy clustering method based on preference information proposed by the present invention, which generally includes the following steps:
[0059] Step A. Input the image to be segmented and set the initial parameter values.
[0060] Input the image I to be divided;
[0061] Set initial parameter values: population size E=50, fuzzy index m=2, maximum iteration number of fine search TF max =50, the threshold σ=0.3, the reference point is (0.1,0.9), the maximum number of iterations of rough search TC max =20, the crossover probability is 0.9, the mutation probability is 0.1, the coefficient β=0.3 to control the dimension of the coarse search space, the weight factor χ=0.5 to control the image region information, and the number of reference vectors v=40;
[0062] Step B: Apply the multi-objective evolutionary fuzzy clustering method based on reference points and preference angles guided by coarse a...
Embodiment 2
[0080] Refer to attached figure 1 , the method proposed by the present invention specifically includes the following steps:
[0081] Step 1: Input the image I to be segmented, and set the initial parameter values: population size E=50, fuzzy index m=2, maximum iteration number TF of fine search max =50, the threshold σ=0.3, the reference point is (0.1,0.9), the maximum number of iterations of rough search TC max =20, the crossover probability is 0.9, the mutation probability is 0.1, the coefficient β=0.3 to control the dimension of the coarse search space, the weight factor χ=0.5 to control the image region information, and the number of reference vectors v=40;
[0082] Step 2: Use Latin hypercube sampling to obtain the initial population X, and encode the chromosomes in the population;
[0083] Step 3: Decode each encoded chromosome to obtain a column vector, calculate the fitness function value Y corresponding to each chromosome, specifically calculate the global fuzzy com...
PUM
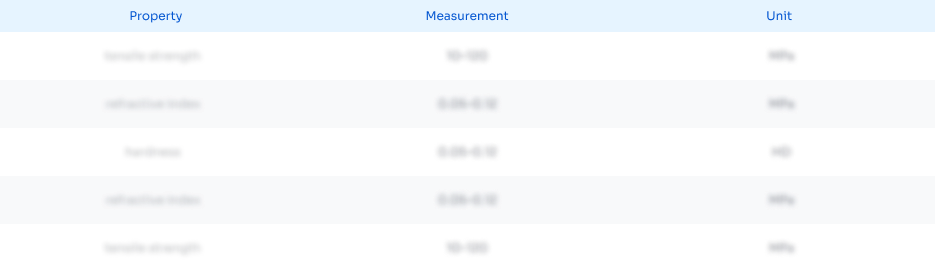
Abstract
Description
Claims
Application Information
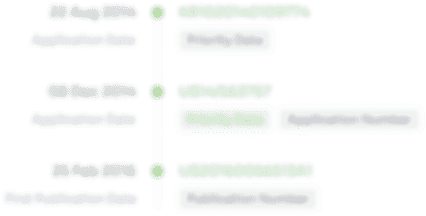
- R&D Engineer
- R&D Manager
- IP Professional
- Industry Leading Data Capabilities
- Powerful AI technology
- Patent DNA Extraction
Browse by: Latest US Patents, China's latest patents, Technical Efficacy Thesaurus, Application Domain, Technology Topic, Popular Technical Reports.
© 2024 PatSnap. All rights reserved.Legal|Privacy policy|Modern Slavery Act Transparency Statement|Sitemap|About US| Contact US: help@patsnap.com