Multi-organ segmentation method based on self-supervised feature small sample learning
A small-sample, multi-organ technology, applied in the field of image processing, can solve problems such as support set supervision information is difficult to fully guide query set segmentation, shape and size information is less, and small-sample learning can not get good results, so as to reduce the interference of recognition , Improve recognition ability, strengthen learning effect
- Summary
- Abstract
- Description
- Claims
- Application Information
AI Technical Summary
Problems solved by technology
Method used
Image
Examples
Embodiment Construction
[0029] The embodiments and effects of the present invention will be further described in detail with reference to the accompanying drawings.
[0030] refer to figure 1 , the implementation steps of this example include the following:
[0031] Step 1, using the superpixel segmentation method to generate a training set and a test set for small sample learning from the original data.
[0032] The training set and test set data of small sample learning are divided into tasks, and the data of each task includes support set and query set. Specifically, in the problem of multi-organ segmentation, each task is actually the segmentation of a single organ, and the training tasks transformed from single-organ segmentation are few, which cannot meet the training needs of small-sample learning methods. To solve this problem, the specific implementation of this step is as follows:
[0033] 1.1) Using the unsupervised training data category generation method based on superpixel segmentati...
PUM
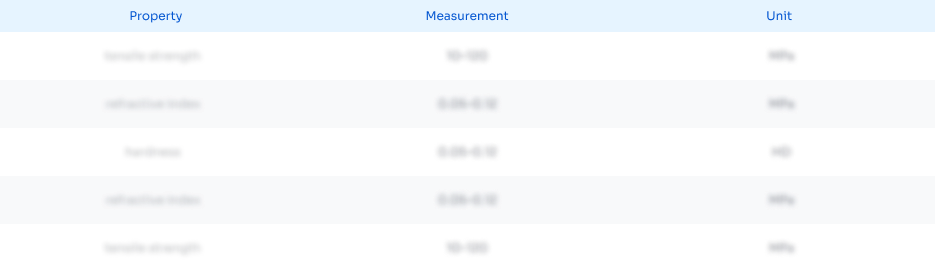
Abstract
Description
Claims
Application Information
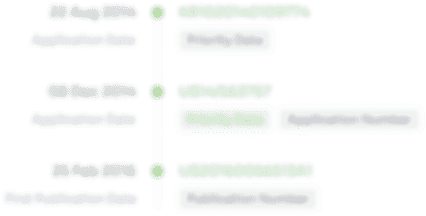
- R&D Engineer
- R&D Manager
- IP Professional
- Industry Leading Data Capabilities
- Powerful AI technology
- Patent DNA Extraction
Browse by: Latest US Patents, China's latest patents, Technical Efficacy Thesaurus, Application Domain, Technology Topic, Popular Technical Reports.
© 2024 PatSnap. All rights reserved.Legal|Privacy policy|Modern Slavery Act Transparency Statement|Sitemap|About US| Contact US: help@patsnap.com