View-level text sentiment classification system and method based on a graph convolutional neural network
A convolutional neural network and emotion classification technology, applied in the field of view-level text emotion classification method and system, can solve the problems of inability to accurately express the true emotion of the text, the inability to distinguish the emotional polarity of comments, and low pixels, so as to achieve good text emotion features Expressed effect
- Summary
- Abstract
- Description
- Claims
- Application Information
AI Technical Summary
Problems solved by technology
Method used
Image
Examples
Embodiment Construction
[0060] The present invention will be further described below in conjunction with the accompanying drawings and embodiments.
[0061] Please refer to figure 1 , the present invention provides a view-level text sentiment classification system based on a graph convolutional neural network, including:
[0062] A text preprocessing module for characterizing view-level text;
[0063] The text semantic information acquisition module is used to capture the bidirectional semantic dependencies of the text;
[0064] Attention encoding module, which is used to capture the global internal correlation of text word sequences and perform further information integration;
[0065] The graph convolutional neural network module applies GCN directly to the sentence dependency tree to model the sentence structure, which can propagate context and dependency information from viewpoint words to viewpoint words;
[0066] The sentiment category output module uses the classification function to obtai...
PUM
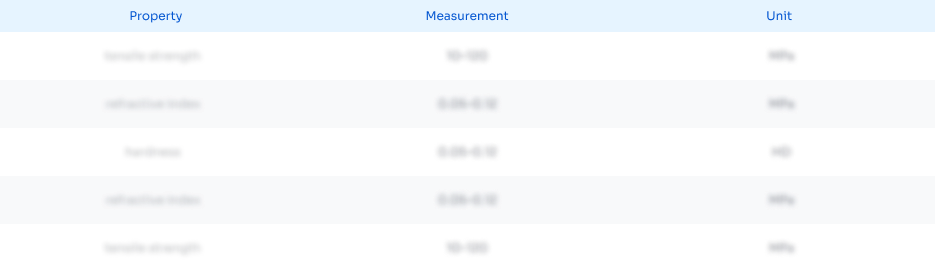
Abstract
Description
Claims
Application Information
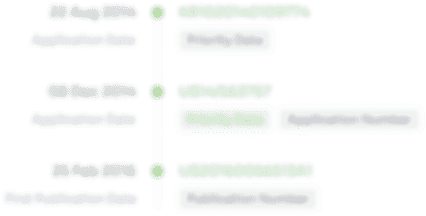
- R&D Engineer
- R&D Manager
- IP Professional
- Industry Leading Data Capabilities
- Powerful AI technology
- Patent DNA Extraction
Browse by: Latest US Patents, China's latest patents, Technical Efficacy Thesaurus, Application Domain, Technology Topic.
© 2024 PatSnap. All rights reserved.Legal|Privacy policy|Modern Slavery Act Transparency Statement|Sitemap