Human body behavior recognition method based on multi-class spectrograms and composite convolutional neural network
A neural network and recognition method technology, applied in the field of human behavior recognition, can solve the problems of incomplete feature extraction, low efficiency of manual feature extraction, single feature expression, etc., and achieve the effect of overcoming low feature efficiency
- Summary
- Abstract
- Description
- Claims
- Application Information
AI Technical Summary
Problems solved by technology
Method used
Image
Examples
Embodiment 1
[0055] Embodiment 1: see Figure 1-Figure 3 , a human behavior recognition method based on a multi-class spectrogram and a composite convolutional neural network, comprising the following steps;
[0056] (1) Sampling different human behaviors n times by stepping frequency continuous wave radar, recording the human behavior category of each sampling, and obtaining a human behavior sample each time, the sample is a matrix of N×M, where N is the number of pulse periods, M is the number of stepping frequencies in a pulse period, and a data set X is obtained by sampling n times,
[0057] X={X i ∈ R N×M |i=1,2,...,n}
[0058] The R is a complex number, N×M is the matrix dimension, X i is the i-th sample in X; the human behavior categories include jumping, picking up things, running, squatting, stepping, arm swinging, throwing and walking;
[0059] (2) Each X i Select S column vectors in , and get n×S column vectors;
[0060] (3) Construct a spectrogram sample data set, includ...
Embodiment 2
[0076] Example 2: see Figure 1 to Figure 7 , in order to better illustrate the effect of the present invention, we specifically set as follows:
[0077] (1) Before performing different human behaviors through step frequency continuous wave radar, the following experimental settings are determined first:
[0078] Radar parameters: the radar frequency range is 1.6GHz to 2.2GHz, the power is 20dBm, the pulse period is 30ms, and in one pulse period, the initial frequency is f 0 , the number of steps is 300 times, and the step frequency increment is 2MHz, that is to say: the pulse period △t=30ms, within one pulse period, the number of step frequencies is N=301, and the frequency is from f 0 , f 0 +2MHz, ..., f 0 +300×2MHz changes in turn, and within 30ms of a pulse period, the radar frequency completes a frequency cycle from 1.6GHz to 2.2GHz. In addition, in this embodiment, a stepped frequency continuous wave radar with two transmissions and four receptions is adopted.
[00...
Embodiment 3
[0090] Embodiment 3: In the present embodiment, the short-time Fourier transform of step (31), the Hanning kernel reduces cross-term interference distribution, and the smooth pseudo-Wigner distribution carries out time-frequency analysis specifically as follows:
[0091]
[0092] In the formula, STFTs(k, f) means: the result of short-time Fourier transform on the original signal; s(·) means: the original signal; k means: time variable; e is a natural constant; f is frequency; w(· ) represents the window function; u represents the convolution variable.
[0093]
[0094] Among them, R s for:
[0095]
[0096] RIDHKs (k, f) represents: carry out Hanning kernel of the present invention to the original signal and reduce the result of cross-term interference distribution transformation;
[0097] s( ) means: original signal;
[0098] R s (k, τ) means: the result of adding a time window to the original signal;
[0099] h(τ) means: frequency window function;
[0100] f m...
PUM
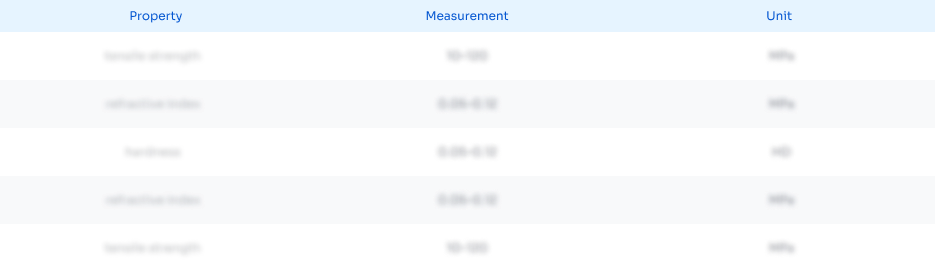
Abstract
Description
Claims
Application Information
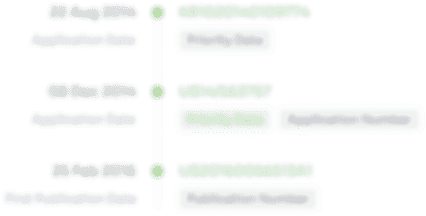
- Generate Ideas
- Intellectual Property
- Life Sciences
- Materials
- Tech Scout
- Unparalleled Data Quality
- Higher Quality Content
- 60% Fewer Hallucinations
Browse by: Latest US Patents, China's latest patents, Technical Efficacy Thesaurus, Application Domain, Technology Topic, Popular Technical Reports.
© 2025 PatSnap. All rights reserved.Legal|Privacy policy|Modern Slavery Act Transparency Statement|Sitemap|About US| Contact US: help@patsnap.com