Disordered grabbing multi-objective optimization method and system based on deep reinforcement learning
A technology of multi-objective optimization and reinforcement learning, applied in the field of multi-objective optimization of disordered grasping based on deep reinforcement learning, to achieve the effect of optimal selection
- Summary
- Abstract
- Description
- Claims
- Application Information
AI Technical Summary
Problems solved by technology
Method used
Image
Examples
Embodiment 1
[0061] Such as figure 1 As shown, this embodiment 1 provides a multi-objective optimization method for out-of-order grasping based on deep reinforcement learning. Through two parallel and independent Q-networks, and processing the same scene at the same time, the robotic arm grasps the two networks respectively. Fetch points to perform capture, and returns parameters such as the execution path and capture power consumption. The Q-network will distinguish the advantages and disadvantages of the two in terms of execution path, capture power consumption, etc., and generate corresponding reward values. The Q network accepts both internal and external reward function feedback, which solves the problem that the reward value function of a single Q network can only be discrete data, and adds continuous data such as execution path and power consumption to the reward value function to further optimize Selection of grab points.
[0062] Specifically, the multi-objective optimization me...
Embodiment 2
[0102] see figure 2 , this embodiment provides a disordered grasping multi-objective optimization system based on deep reinforcement learning, the system includes: a virtual scene construction module, a task establishment module, a virtual shooting module, an output module, an execution module, a calculation module, a feedback module and the predictive model generation module.
[0103] The virtual scene building module is suitable for constructing a virtual scene in which a robotic arm grabs multiple objects.
[0104] The task establishment module is suitable for establishing two parallel and independent deep reinforcement learning networks to handle the task of grabbing multiple targets out of order. Specifically, the task building module is used to perform the following steps:
[0105] S121: Establish two parallel and independent deep reinforcement learning networks, namely a first network and a second network, wherein the first network and the second network have the sam...
Embodiment 3
[0136] This embodiment provides a computer-readable storage medium, and at least one instruction is stored in the computer-readable storage medium. When the above-mentioned instruction is executed by a processor, the disorder based on deep reinforcement learning provided by Embodiment 1 is realized. Catch multi-objective optimization methods.
[0137] The multi-objective optimization method for out-of-order grasping based on deep reinforcement learning uses two parallel and independent Q-networks to process the same scene at the same time. The robotic arm performs grasping on the respective grasping points of the two networks and returns the execution path. Capture parameters such as power consumption. The Q-network will distinguish the advantages and disadvantages of the two in terms of execution path, capture power consumption, etc., and generate corresponding reward values. The Q network accepts both internal and external reward function feedback, which solves the problem ...
PUM
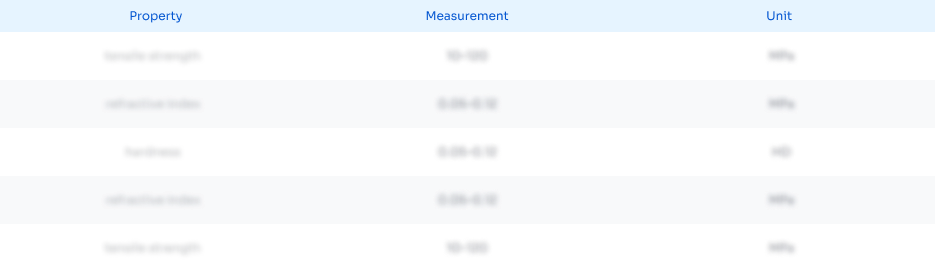
Abstract
Description
Claims
Application Information
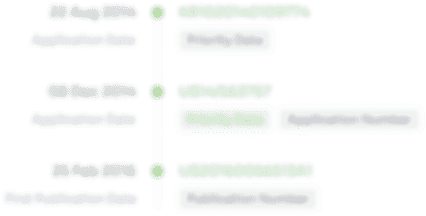
- R&D Engineer
- R&D Manager
- IP Professional
- Industry Leading Data Capabilities
- Powerful AI technology
- Patent DNA Extraction
Browse by: Latest US Patents, China's latest patents, Technical Efficacy Thesaurus, Application Domain, Technology Topic, Popular Technical Reports.
© 2024 PatSnap. All rights reserved.Legal|Privacy policy|Modern Slavery Act Transparency Statement|Sitemap|About US| Contact US: help@patsnap.com