Cluster energy prediction method and system
An energy prediction and clustering technology, applied in neural learning methods, neural architectures, biological neural network models, etc., can solve the problems of complex clusters, the lowest energy in searching for the global optimal structure of clusters, and the increase in computing time, etc. Prediction accuracy, the effect of improving energy prediction accuracy
- Summary
- Abstract
- Description
- Claims
- Application Information
AI Technical Summary
Problems solved by technology
Method used
Image
Examples
Embodiment 1
[0041] Such as Figure 1-3 As shown, Embodiment 1 of the present disclosure provides a cluster energy prediction method for non-metallic clusters, including the following process:
[0042] Obtain all atomic coordinates of non-metallic clusters, expand the acquired atomic coordinates, and preprocess the expanded atomic coordinate data;
[0043] According to the preprocessed atomic coordinate data and the preset multi-layer neural network model, the energy prediction results of non-metallic clusters are obtained;
[0044] Among them, the mean square error loss function is used in the preset multi-layer neural network model.
[0045] Specifically, include the following:
[0046] It is necessary to analyze according to the characteristics of non-metallic clusters, use the MLP neural network to model, expand the (x, y, z) coordinates of all atoms, regard each coordinate as a feature value, and use the final energy as the target value to carry out MLP Regression Prediction with N...
Embodiment 2
[0102] Embodiment 2 of the present disclosure provides a cluster energy prediction system, including:
[0103] The data preprocessing module is configured to: obtain all atomic coordinates of the non-metallic cluster, expand the acquired atomic coordinates, and preprocess the expanded atomic coordinate data;
[0104] The energy prediction module is configured to: obtain the energy prediction result of the non-metallic cluster according to the preprocessed atomic coordinate data and the preset multi-layer neural network model;
[0105] Among them, the mean square error loss function is used in the preset multi-layer neural network model.
[0106] The working method of the system is the same as the cluster energy prediction method provided in Embodiment 1, and will not be repeated here.
Embodiment 3
[0108] Embodiment 3 of the present disclosure provides a computer-readable storage medium on which a program is stored, and when the program is executed by a processor, the steps in the cluster energy prediction method described in Embodiment 1 of the present disclosure are implemented.
PUM
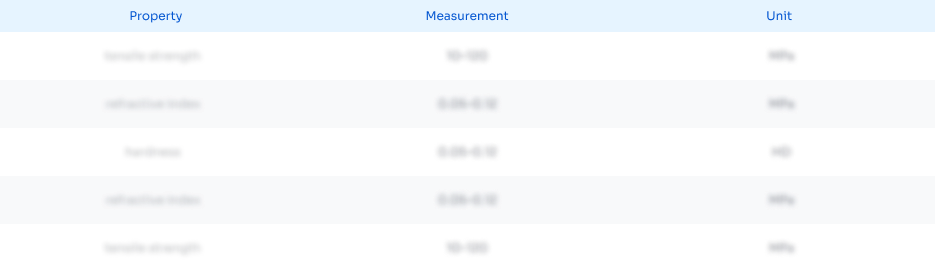
Abstract
Description
Claims
Application Information
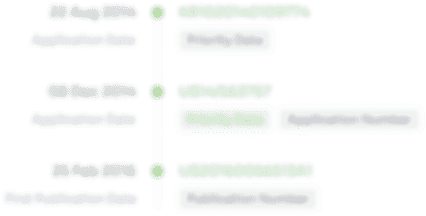
- R&D Engineer
- R&D Manager
- IP Professional
- Industry Leading Data Capabilities
- Powerful AI technology
- Patent DNA Extraction
Browse by: Latest US Patents, China's latest patents, Technical Efficacy Thesaurus, Application Domain, Technology Topic, Popular Technical Reports.
© 2024 PatSnap. All rights reserved.Legal|Privacy policy|Modern Slavery Act Transparency Statement|Sitemap|About US| Contact US: help@patsnap.com