Bond market default risk early warning method based on interpretable machine learning
A machine learning and risk early warning technology, applied in the field of default risk early warning, can solve problems such as depression, inability to effectively predict minority groups, and unbalanced debt default risk prediction categories, and achieve the effect of making up for low interpretability
- Summary
- Abstract
- Description
- Claims
- Application Information
AI Technical Summary
Problems solved by technology
Method used
Image
Examples
Embodiment
[0053] The bond market default risk early warning method based on explainable machine learning includes the following steps:
[0054] S1. Obtain data, obtain past quarterly / annual financial data, corporate governance, company characteristics, and measurement variables of the market environment of companies that have defaulted on bonds for the first time through market research, and select existing corporate bonds, corporate bonds, or medium-term bonds in the same period Companies that have not defaulted on their bonds and have not defaulted on their bonds are used as non-default samples;
[0055] S2. Data preprocessing, first sorting out the data, then extracting various types of data of debt default enterprises, and performing variable screening on them, and at the same time dividing the data set;
[0056] S3. Select a model and construct a model, select an existing model as a comparison, and simultaneously establish a variety of machine learning models;
[0057] S4. Model t...
PUM
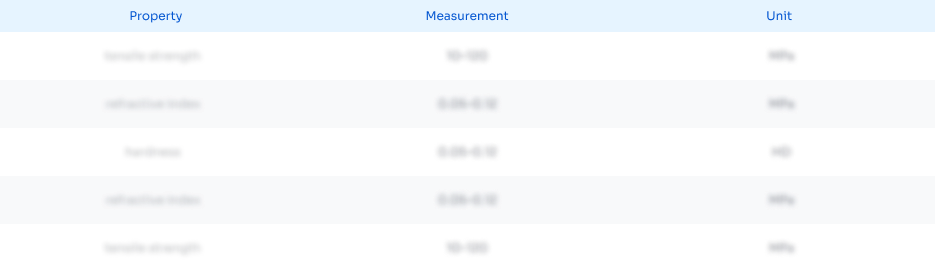
Abstract
Description
Claims
Application Information
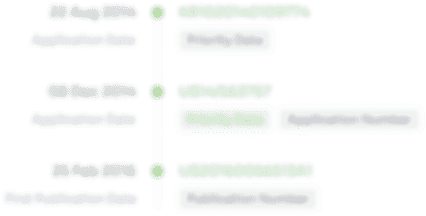
- R&D Engineer
- R&D Manager
- IP Professional
- Industry Leading Data Capabilities
- Powerful AI technology
- Patent DNA Extraction
Browse by: Latest US Patents, China's latest patents, Technical Efficacy Thesaurus, Application Domain, Technology Topic, Popular Technical Reports.
© 2024 PatSnap. All rights reserved.Legal|Privacy policy|Modern Slavery Act Transparency Statement|Sitemap|About US| Contact US: help@patsnap.com