OTD_Loglogistic-based SAR (Synthetic Aperture Radar) data ocean target detection method
A technology for target detection and detection methods, applied in neural learning methods, ICT adaptation, biological neural network models, etc., can solve problems that are not scientific and universal, no longer conform to Gaussian distribution, and complex sea clutter distribution. Achieve the effect of overcoming the disaster of dimensionality, overcoming the long computational cost, and improving the detection efficiency and accuracy
- Summary
- Abstract
- Description
- Claims
- Application Information
AI Technical Summary
Problems solved by technology
Method used
Image
Examples
Embodiment Construction
[0039] Below in conjunction with accompanying drawing and specific embodiment the present invention is described in further detail:
[0040] 1. Lightweight deep learning model construction
[0041] 1. OceanTDAx series model construction
[0042] When building a lightweight deep learning model, the OceanTDAx series, a lightweight convolutional neural network model, was first designed. The OceanTDAx series includes four models, namely OceanTDA2, OceanTDA4, OceanTDA9, and OceanTDA16. The OceanTDA9 model has the best detection effect in preliminary experiments.
[0043] The structure of the OceanTDA9 model is as follows figure 1 As shown, it contains 4 convolutional layers, 1 convolutional group and 3 fully connected layers. The first 4 convolutional layers are Conv2D_1, Conv2D_2, Conv2D_3, Conv2D_4. The form of each convolution is the same, which is Convolution2D- ReLU-Dropout-Maxpooling; the middle convolution group is Conv2D_g, and the organization form is (Convolution2D-ReL...
PUM
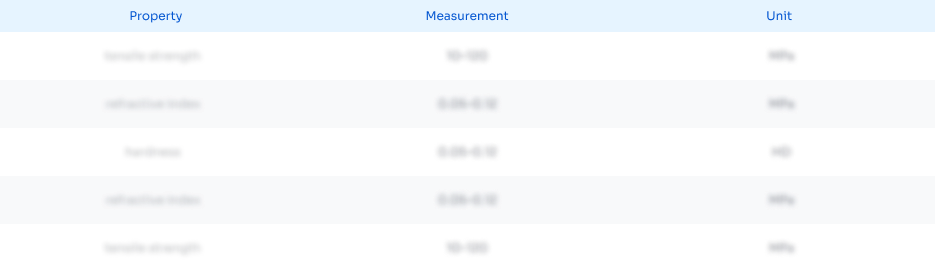
Abstract
Description
Claims
Application Information
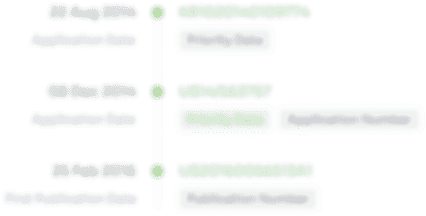
- R&D Engineer
- R&D Manager
- IP Professional
- Industry Leading Data Capabilities
- Powerful AI technology
- Patent DNA Extraction
Browse by: Latest US Patents, China's latest patents, Technical Efficacy Thesaurus, Application Domain, Technology Topic, Popular Technical Reports.
© 2024 PatSnap. All rights reserved.Legal|Privacy policy|Modern Slavery Act Transparency Statement|Sitemap|About US| Contact US: help@patsnap.com