Prediction result identification method, prediction result identification model training method, prediction result identification model training device and computer storage medium
A technology for predicting results and identification, applied in the field of sample identification, can solve the problems of increasing false positives, low-quality positive and negative samples, and small target positive samples, and achieves the effect of avoiding cold start and improving training performance.
- Summary
- Abstract
- Description
- Claims
- Application Information
AI Technical Summary
Problems solved by technology
Method used
Image
Examples
no. 1 example
[0033] figure 1 The processing flow of the prediction result identification method in the first embodiment of the present application is shown. As shown in the figure, the prediction result identification method in this embodiment mainly includes:
[0034] Step S102, according to the sample picture, determine the category label and position label of at least one labeled box in the sample picture, and obtain the prediction results of each anchor box in the sample picture.
[0035] In this embodiment, the label frame is used to identify at least one target object in the sample picture; the category label of the label frame is used to identify the category of the target object (for example: people, animals, plants, buildings, etc.); the sample picture The location label is used to identify the location of each object in the sample image.
[0036] In this embodiment, the reference model is a picture recognition model with a picture recognition function.
[0037] In this embodime...
no. 2 example
[0048] figure 2 The processing flow of the prediction result identification method according to the second embodiment of the present application is shown. This embodiment mainly shows the specific implementation scheme of the first sample identification. As shown in the figure, the prediction result identification method of this embodiment mainly includes:
[0049] Step S202, according to each position prediction information of each anchor frame and the position label of the annotation frame, determine each anchor frame falling into the annotation frame as each candidate anchor frame.
[0050] In this embodiment, when there are multiple annotation frames in the sample picture (that is, when there are multiple objects in the sample image), one annotation frame is selected in turn, and each anchor frame is compared with the currently selected annotation frame respectively. Perform comparative analysis.
[0051] In this embodiment, each anchor frame falling into the annotation...
no. 3 example
[0064] image 3 It shows the processing flow of the prediction result identification method of the third embodiment of the present application. This embodiment shows the specific implementation scheme of the second sample identification. As shown in the figure, the prediction result identification method of this embodiment mainly includes:
[0065] Step S302, according to each position prediction information of each anchor frame and the position label of the annotation frame, each intersection ratio of each anchor frame relative to the annotation frame is obtained.
[0066] In this embodiment, according to the location prediction information of each anchor frame and the location label of the annotation frame, the intersection ratio between all the anchor frames and the annotation frame can be calculated.
[0067] Step S304: According to each intersection and union ratio of each anchor box and a preset threshold, the anchor frame prediction results of each anchor frame whose in...
PUM
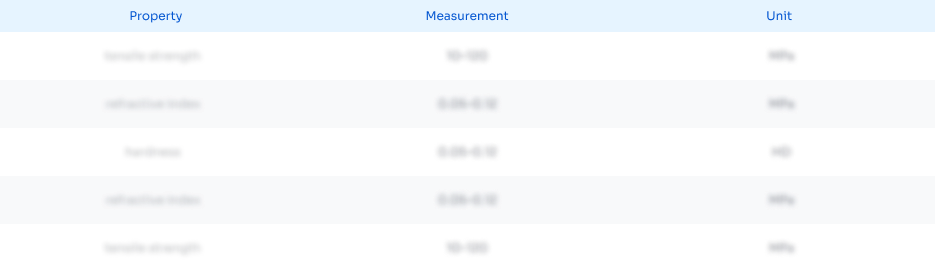
Abstract
Description
Claims
Application Information
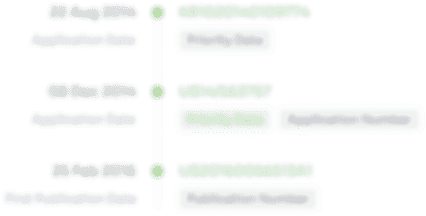
- R&D Engineer
- R&D Manager
- IP Professional
- Industry Leading Data Capabilities
- Powerful AI technology
- Patent DNA Extraction
Browse by: Latest US Patents, China's latest patents, Technical Efficacy Thesaurus, Application Domain, Technology Topic, Popular Technical Reports.
© 2024 PatSnap. All rights reserved.Legal|Privacy policy|Modern Slavery Act Transparency Statement|Sitemap|About US| Contact US: help@patsnap.com