MAC protocol for realizing time domain interference alignment based on deep reinforcement learning in underwater acoustic network
A technology of reinforcement learning and underwater acoustic network, applied in wireless network protocol, network topology, ultrasonic/acoustic/infrasonic transmission system, etc., can solve the problems of algorithm complexity increase and algorithm calculation infeasibility, and achieve the effect of improving throughput
- Summary
- Abstract
- Description
- Claims
- Application Information
AI Technical Summary
Problems solved by technology
Method used
Image
Examples
example
[0058] In order to verify the performance of the DQNSA-MAC protocol of the present invention, carry out following simulation experiments:
[0059] The DQNSA-MAC protocol is implemented and simulated based on the TensorFlow framework using the Python programming language. In the DQNSA-MAC protocol, DQN includes a 100-unit LSTM layer. The minibatch size is set to 200episode, and the step size of each episode is 50. The discount factor was set to γ=0.9. We trained this network for more than 10,000 iterations, and compared the DQNSA-MAC protocol with slotted-Aloha and DPSA protocols in terms of throughput, message forwarding success rate, and fairness index.
[0060] The success rate of message forwarding (SDR) refers to the number of messages successfully received (M received ) and the total number of messages generated in the network (M generated ) ratio, as shown in formula (10). In order to evaluate the fairness of the underlying protocol, we use Jain’s FairnessIndex, as ...
PUM
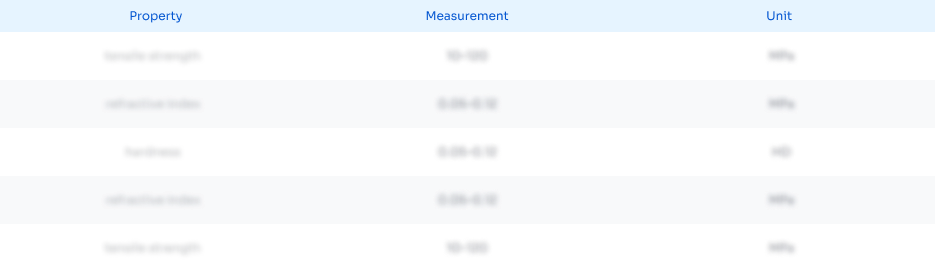
Abstract
Description
Claims
Application Information
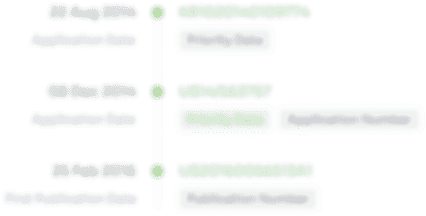
- R&D Engineer
- R&D Manager
- IP Professional
- Industry Leading Data Capabilities
- Powerful AI technology
- Patent DNA Extraction
Browse by: Latest US Patents, China's latest patents, Technical Efficacy Thesaurus, Application Domain, Technology Topic, Popular Technical Reports.
© 2024 PatSnap. All rights reserved.Legal|Privacy policy|Modern Slavery Act Transparency Statement|Sitemap|About US| Contact US: help@patsnap.com