Real image denoising method based on multi-scale selection feedback network
A feedback network, real image technology, applied in the field of computer vision and image processing, can solve the problems of robustness to noise changes, reducing over-reliance on clean and high-quality training data, and high complexity of denoising models
- Summary
- Abstract
- Description
- Claims
- Application Information
AI Technical Summary
Problems solved by technology
Method used
Image
Examples
Embodiment Construction
[0021] The present invention will be further described below in conjunction with the accompanying drawings and specific embodiments.
[0022] Embodiments of the present invention propose a real image denoising method based on a multi-scale selective feedback network, which mainly includes steps S1-S5:
[0023] S1. Construct a multi-scale selection block (multi-scale selection block, MSB) for extracting multiple receptive field scale features.
[0024] figure 2 is a schematic diagram of the multi-scale selection module of the embodiment of the present invention. like figure 2 As shown, the multi-scale selection module (MSB) includes a feature extraction unit 10, a feature compression unit 20, a feature importance probability distribution unit 30, a feature calibration unit 40 and a fusion output unit 50 connected in sequence from the input end to the output end. exist figure 2 In the exemplary network shown, the feature extraction unit 10 uses three parallel convolutiona...
PUM
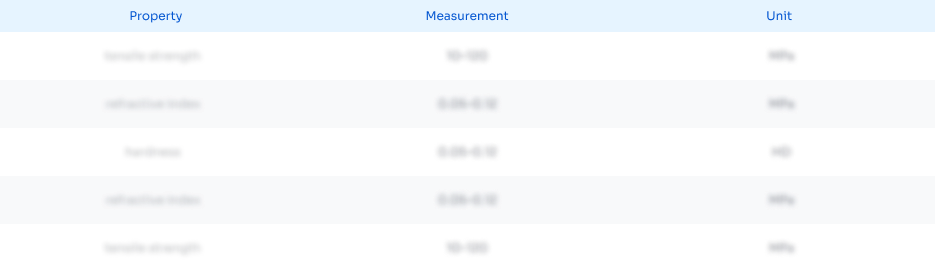
Abstract
Description
Claims
Application Information
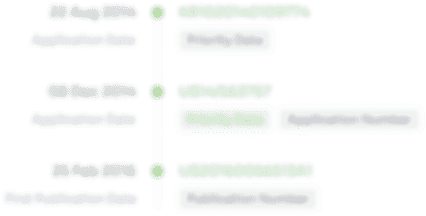
- R&D Engineer
- R&D Manager
- IP Professional
- Industry Leading Data Capabilities
- Powerful AI technology
- Patent DNA Extraction
Browse by: Latest US Patents, China's latest patents, Technical Efficacy Thesaurus, Application Domain, Technology Topic, Popular Technical Reports.
© 2024 PatSnap. All rights reserved.Legal|Privacy policy|Modern Slavery Act Transparency Statement|Sitemap|About US| Contact US: help@patsnap.com