Rapid DRBM adaptation method based on meta-learning
A meta-learning and fast technology, applied in the field of machine learning, can solve the problems of DRBM network underfitting, the initial value of network parameters cannot reach the global optimum, etc.
- Summary
- Abstract
- Description
- Claims
- Application Information
AI Technical Summary
Problems solved by technology
Method used
Image
Examples
example 1
[0083] In Example 1, the HRRP simulation data output by the three-dimensional aircraft model electromagnetic simulation software designed by Saibo Company is used. The three types of aircraft simulated are F-35, F-117 and P-51. The specific parameters of the aircraft are as follows: Figure 4 shown.
[0084] The radar simulation band is x-band. The frequency range is 9.5GHz-10.5GHz with a step size of 5MHz. The polarization mode is vertical polarization. The pitch angle of the target is 0°~10° with a step size of 0.1°, and the azimuth angle is 0°~90° with a step size of 0.1°. Therefore, our data set has a total of 201 frequency points, 101 elevation angles and 901 azimuth angles, that is, a total of 101×901=91001 samples, and each sample has 201 dimensions.
[0085] Figure 5It shows the changes of HRRP data during preprocessing, where the x-axis represents the data dimension and the y-axis represents the signal amplitude. (a) is the HRRP data before preprocessing, (b) is...
example 2
[0103] In Example 2, the handwritten digits (MNIST) dataset was used for experiments. The MNIST data set consists of 70,000 pictures and corresponding labels, of which 60,000 are used to train the neural network and 10,000 are used to test the neural network. Each picture is a handwritten digital picture of 0-9 with 28*28 pixels. The picture is white on a black background. Black is represented by 0, and white is represented by a floating point number between 0 and 1. The closer to 1, the whiter the color. The MNIST data set provides the label corresponding to each picture, which is given in one-hot form, that is, the label vector is a one-dimensional array with a length of 10. A sample of the MNIST dataset such as Figure 8 shown.
[0104] We form 784 pixels into a one-dimensional array with a length of 784. This one-dimensional array is the input data we want to input into the neural network. For the RBM network, the input data of the network can only be binary, so the MN...
PUM
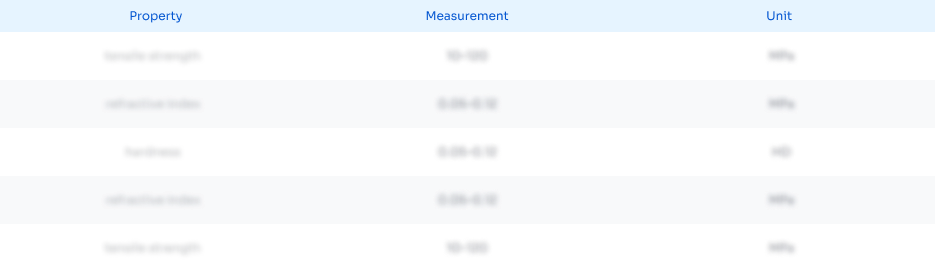
Abstract
Description
Claims
Application Information
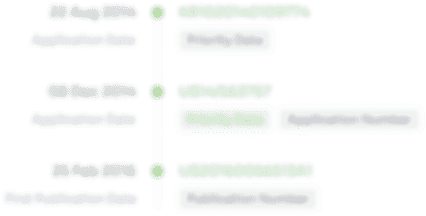
- Generate Ideas
- Intellectual Property
- Life Sciences
- Materials
- Tech Scout
- Unparalleled Data Quality
- Higher Quality Content
- 60% Fewer Hallucinations
Browse by: Latest US Patents, China's latest patents, Technical Efficacy Thesaurus, Application Domain, Technology Topic, Popular Technical Reports.
© 2025 PatSnap. All rights reserved.Legal|Privacy policy|Modern Slavery Act Transparency Statement|Sitemap|About US| Contact US: help@patsnap.com