Hyperspectral image target detection method based on sample mining and background reconstruction
A hyperspectral image and target detection technology, applied in neural learning methods, scene recognition, neural architecture, etc., can solve the problems of insufficient utilization of hyperspectral image background information, insufficient background interference suppression, insufficient effective training samples, etc. Achieve the effect of improving training effect and detection accuracy, suppressing background interference, and removing noise interference
- Summary
- Abstract
- Description
- Claims
- Application Information
AI Technical Summary
Problems solved by technology
Method used
Image
Examples
Embodiment Construction
[0037] The embodiments and effects of the present invention will be further described in detail below in conjunction with the accompanying drawings.
[0038] refer to figure 1 , the implementation steps of this example are as follows.
[0039] Step 1. Obtain the hyperspectral image X to be detected and the real spectral vector d of the target to be detected.
[0040] Select the hyperspectral image X to be detected with a size of M×N×L from the hyperspectral image library, and the real spectral vector d similar to the spectral curve of the target to be detected contained in the hyperspectral image X to be detected, where M, N , L are the width, height, and number of spectral bands of the hyperspectral image X to be detected, respectively, M>0, N>0, L≥100; in this example, the hyperspectral image X to be detected is collected by the ROSIS sensor of the reflective optical system imaging spectrometer The real hyperspectral image of , which has 102 spectral bands, has a size of 1...
PUM
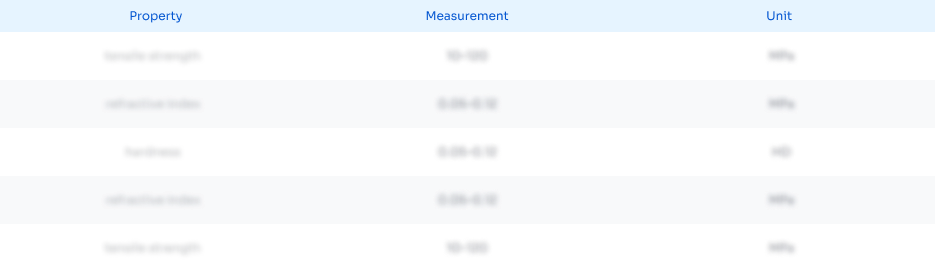
Abstract
Description
Claims
Application Information
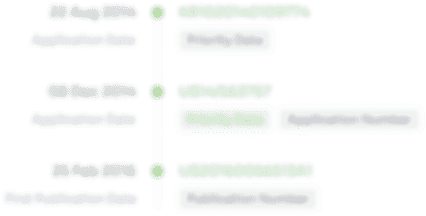
- R&D
- Intellectual Property
- Life Sciences
- Materials
- Tech Scout
- Unparalleled Data Quality
- Higher Quality Content
- 60% Fewer Hallucinations
Browse by: Latest US Patents, China's latest patents, Technical Efficacy Thesaurus, Application Domain, Technology Topic, Popular Technical Reports.
© 2025 PatSnap. All rights reserved.Legal|Privacy policy|Modern Slavery Act Transparency Statement|Sitemap|About US| Contact US: help@patsnap.com