Abdominal lymph node partitioning method based on attention mechanism neural network
A neural network and attention technology, applied in neural learning methods, biological neural network models, computer components, etc., can solve problems such as large differences in reading results and inaccurate prediction of abdominal lymph node divisions.
- Summary
- Abstract
- Description
- Claims
- Application Information
AI Technical Summary
Problems solved by technology
Method used
Image
Examples
Embodiment
[0062] Such as figure 1 As shown, an abdominal lymph node segmentation method based on the attention mechanism neural network includes the following steps:
[0063] Step 1: Data preparation, completing data import from the data system and calibration of abdominal lymph nodes to be classified;
[0064] Step 2: Mask generation, preprocessing the data, mainly including the preprocessing of the original CT image and using different strategies to generate the mask of the lymph node region;
[0065] Step 3: Build an attention mechanism residual network model, and use the collected data and calibration results to train the model;
[0066] Step 4: Repeat step 3 to build and train a model for the relative position and partition of abdominal lymph nodes; the "relative position" here refers to the position of abdominal lymph nodes in the abdominal structure relative to the complicated tissues such as organs and blood vessels, and the "partition" is defined by the location of lymph node...
PUM
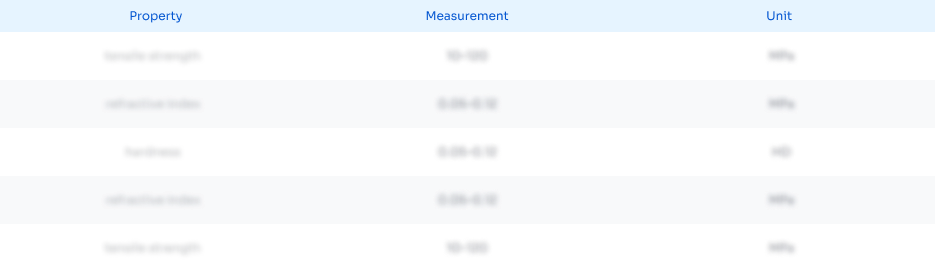
Abstract
Description
Claims
Application Information
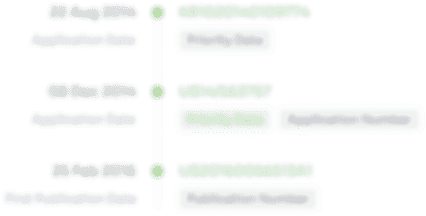
- R&D Engineer
- R&D Manager
- IP Professional
- Industry Leading Data Capabilities
- Powerful AI technology
- Patent DNA Extraction
Browse by: Latest US Patents, China's latest patents, Technical Efficacy Thesaurus, Application Domain, Technology Topic, Popular Technical Reports.
© 2024 PatSnap. All rights reserved.Legal|Privacy policy|Modern Slavery Act Transparency Statement|Sitemap|About US| Contact US: help@patsnap.com