DDADSM-based cross-subject transfer learning electroencephalogram mental state detection method
A mental state and transfer learning technology, applied in the field of EEG signal recognition, can solve problems such as poor detection effect, low recognition rate, and inability to overcome the loss of projection space offset, so as to improve learning ability and reduce mapping deviation.
- Summary
- Abstract
- Description
- Claims
- Application Information
AI Technical Summary
Problems solved by technology
Method used
Image
Examples
Embodiment Construction
[0095]Further implementation analysis of the present invention will be made in conjunction with specific embodiments.
[0096]The goal of this method is from the source domain DsCharacteristics to learn a classifier F to predict D in the correspondence relationship of the labeltTag of. Taking the "Fatigue" "Fatigue" "Fatigue" "Tired" and "Neutral" three-class mental state as an example, the data used in the experiment is 64 channel eElectronic data, and the two reference electrodes are removed in actual use. Get the 62-channel EEG data.figure 1 For the overall flow chart of the method, the original eElectronic data of multiple fatigue driving experiments was collected under the experimental paradigm of the present invention in accordance with the above-described overall flow, and the original eElectronic data pretreatment was performed in accordance with step (1).figure 2 The 62-channel brain electrical position of the fatigue driving of the present invention is collected. The data uti...
PUM
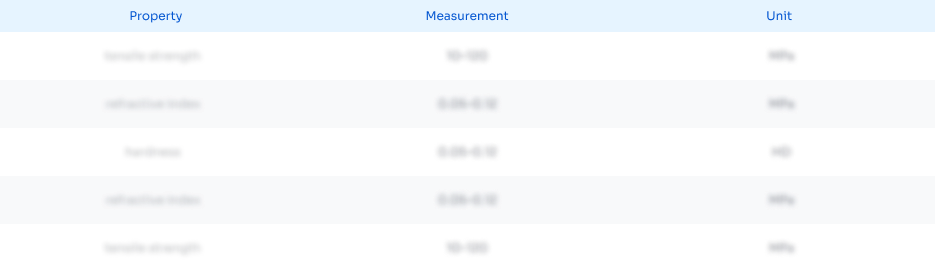
Abstract
Description
Claims
Application Information
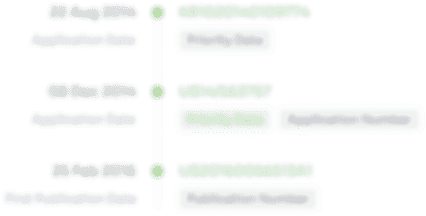
- Generate Ideas
- Intellectual Property
- Life Sciences
- Materials
- Tech Scout
- Unparalleled Data Quality
- Higher Quality Content
- 60% Fewer Hallucinations
Browse by: Latest US Patents, China's latest patents, Technical Efficacy Thesaurus, Application Domain, Technology Topic, Popular Technical Reports.
© 2025 PatSnap. All rights reserved.Legal|Privacy policy|Modern Slavery Act Transparency Statement|Sitemap|About US| Contact US: help@patsnap.com