Kernel extreme learning machine electricity sales prediction method based on generalized maximum correlation entropy criterion
A technology of nuclear extreme learning machine and maximum correlation entropy, which is applied in the direction of neural learning methods, nuclear methods, instruments, etc., can solve the problems of low prediction accuracy of electricity sales and difficulty in meeting the requirements of electricity sales forecast accuracy for electricity sales transactions, etc.
- Summary
- Abstract
- Description
- Claims
- Application Information
AI Technical Summary
Problems solved by technology
Method used
Image
Examples
Embodiment
[0108] Step 1. Apply formula (1) and formula (2) to filter and correct the abnormal data in the historical electricity sales data.
[0109] Step 2. Use an electricity sales company to establish a training sample set for a customer's electricity sales data from January 1, 2018 to May 3, 2018 and the corresponding daily maximum temperature.
[0110] Step 3, apply formula (4) to normalize historical electricity sales data and temperature data.
[0111] Step 4. Use the nuclear extreme learning machine prediction model based on the generalized maximum correlation entropy criterion for training. When training the electricity sales on the tth day, the input of the model is the daily electricity consumption, daily maximum temperature and The daily maximum temperature on day t. Apply formula (13) to update the prediction model parameter β.
[0112] Step 5. Apply formula (11) to obtain the training value of the electricity sold.
[0113] Step 6. Applying the K-fold cross-validation m...
PUM
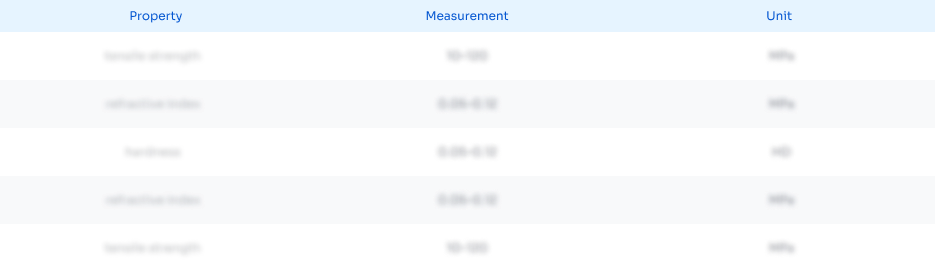
Abstract
Description
Claims
Application Information
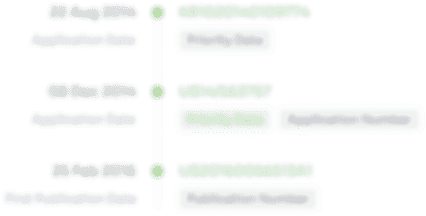
- R&D Engineer
- R&D Manager
- IP Professional
- Industry Leading Data Capabilities
- Powerful AI technology
- Patent DNA Extraction
Browse by: Latest US Patents, China's latest patents, Technical Efficacy Thesaurus, Application Domain, Technology Topic, Popular Technical Reports.
© 2024 PatSnap. All rights reserved.Legal|Privacy policy|Modern Slavery Act Transparency Statement|Sitemap|About US| Contact US: help@patsnap.com