Semi-supervised learning pseudo label assignment method based on clustering fusion
A semi-supervised learning and labeling technology, applied in the field of pseudo-label assignment of semi-supervised learning, can solve the problems of large manpower and financial costs, and achieve the effect of clear principle, easy code, and easy understanding
- Summary
- Abstract
- Description
- Claims
- Application Information
AI Technical Summary
Problems solved by technology
Method used
Image
Examples
Embodiment 1
[0037] refer to Figure 1~2 , which is the first embodiment of the present invention, this embodiment provides a method for assigning pseudo-labels of semi-supervised learning based on cluster fusion, including:
[0038] S1: Construct a feature extraction convolutional neural network, use labeled data and unlabeled data for neural network pre-training, and use the trained network to extract data features. It should be noted that,
[0039] Pre-training the feature extraction convolutional network includes training the resnet101 network with the imagenet database, and then using the pre-trained network to extract the features of all samples in the training data set, and setting the label data feature to f l (x j μ j ), x j is the jth label data, μ j For its corresponding label, the unlabeled data feature is f u (x i ), x i is the i-th unlabeled data.
[0040] S2: Use the nearest neighbor method to assign pseudo-labels to a batch of unlabeled data closest to the labeled ...
Embodiment 2
[0057] In the second embodiment of the present invention, in order to better verify and illustrate the technical effect adopted in the method of the present invention, in this embodiment, the application of pedestrian re-identification is selected for testing, and the test results are compared by means of scientific demonstration. To verify the real effect of the method;
PUM
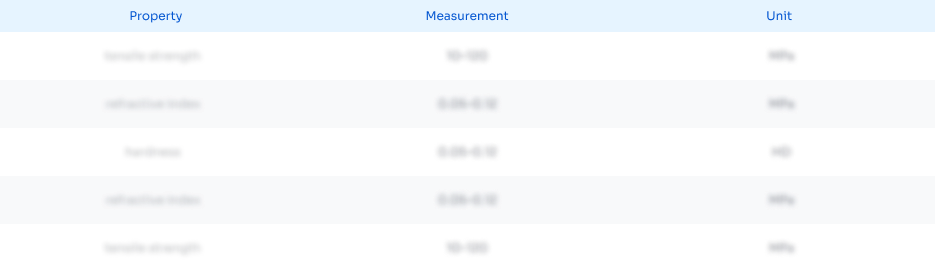
Abstract
Description
Claims
Application Information
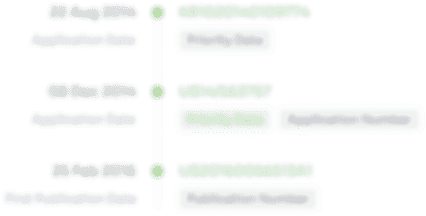
- R&D Engineer
- R&D Manager
- IP Professional
- Industry Leading Data Capabilities
- Powerful AI technology
- Patent DNA Extraction
Browse by: Latest US Patents, China's latest patents, Technical Efficacy Thesaurus, Application Domain, Technology Topic, Popular Technical Reports.
© 2024 PatSnap. All rights reserved.Legal|Privacy policy|Modern Slavery Act Transparency Statement|Sitemap|About US| Contact US: help@patsnap.com