Network structure learning method based on differential privacy
A technology of network structure and differential privacy, which is applied in the field of network structure learning based on differential privacy, can solve problems such as not considering privacy protection, and achieve high availability and privacy protection
- Summary
- Abstract
- Description
- Claims
- Application Information
AI Technical Summary
Problems solved by technology
Method used
Image
Examples
Embodiment 1
[0043] The present invention provides a network structure learning method based on differential privacy, such as figure 1 shown, including the following steps:
[0044] Step 1: Take the network data to be learned as input;
[0045] In reality, a large amount of data does not exist independently, but has a direct or potential network (graph) structure. The main constituent elements of the network are nodes and edges. Nodes are the basic units in a complex system, and edges connect nodes through certain relationships. Use the binary group G=(V, E)G=(V, E) to represent a network (graph), where V=1,...,pV=1,...,p represent node variables P 1 ,...,P p P 1 ,...,P p , Represents the collection of edges, and the network data PP is the node variable P 1 ,...,P p P 1 ,...,P p The specific realization of; for example, in a social network, the node is a certain type of person, and a network is naturally formed between people through a certain relationship; while in a biologic...
Embodiment 2
[0071] On the basis of the network structure learning method based on differential privacy provided in Example 1, this example is specifically based on the gene detection result data, and further explains the above method, specifically as follows figure 1 shown, including the following steps:
[0072] Step 1: Detect genetic data, and form a network dataset based on the genetic detection results;
[0073] This data set is the flow cytometric measurement data of 11 proteins on 7466 cells. The interaction between proteins can naturally form a biological network to infer the potential network structure between proteins. The 11 proteins are 11 network nodes. Flow cytometry measurements on 7466 cells are 7466 samples.
[0074] Step 2: Normalize the network data to form a normalized data matrix;
[0075] In order to give the standard deviation of the Gaussian distribution in step 4, the data matrix needs to be normalized, and the Euclidean norm of each sample is required to be no g...
PUM
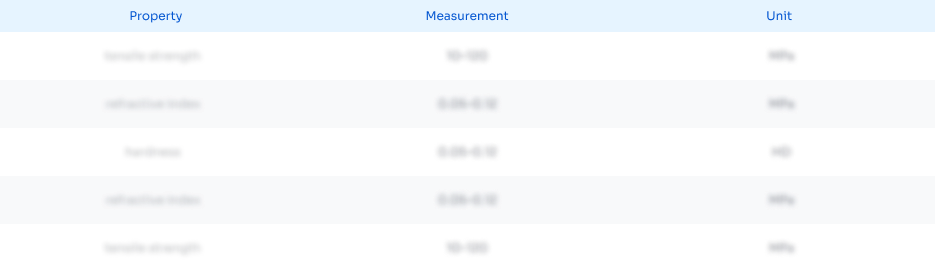
Abstract
Description
Claims
Application Information
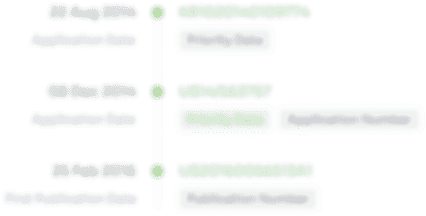
- R&D Engineer
- R&D Manager
- IP Professional
- Industry Leading Data Capabilities
- Powerful AI technology
- Patent DNA Extraction
Browse by: Latest US Patents, China's latest patents, Technical Efficacy Thesaurus, Application Domain, Technology Topic, Popular Technical Reports.
© 2024 PatSnap. All rights reserved.Legal|Privacy policy|Modern Slavery Act Transparency Statement|Sitemap|About US| Contact US: help@patsnap.com