Twin network video target tracking method and device
A twin network and video tracking technology, applied in the field of video analysis, can solve the problems of tracking real-time decline, target drift, etc.
- Summary
- Abstract
- Description
- Claims
- Application Information
AI Technical Summary
Problems solved by technology
Method used
Image
Examples
Embodiment 1
[0089] The present embodiment provides a method for tracking a twin network video object based on a layered attention mechanism. The method includes the following steps:
[0090] Step 1, read and preprocess the template image and search image input by the network;
[0091] Step 2. Input the preprocessed template image and search image into the trained Siamese network model respectively, and obtain the feature maps of the template image and search image respectively through the Inception module and the feature extraction module of the convolutional layer;
[0092] The twin network model is divided into a template branch and a search branch, the template branch and the search branch extract features respectively through the improved feature extraction module, and the attention module is added to the template branch to perform feature recalibration;
[0093] Step 3. Pass the low-level features extracted by the third layer of the template branch network and the high-level features...
Embodiment 2
[0162] This embodiment provides a twin network video target tracking method based on a layered attention mechanism. In order to make the purpose, implementation scheme and advantages of the present invention clearer, the sequence Singer1 in the public test set OTB Benchmark is taken as an example below to describe this The specific implementation of the invention is further described in detail in conjunction with the description of the accompanying drawings, specifically as follows:
[0163]The present invention proposes a Siamese network tracking method based on a hierarchical attention mechanism. This method divides the tracking process into two parts: target position estimation and target scale estimation. Convolutional neural network is used for feature extraction, and the features of the third layer and the features of the fifth layer are weighted and fused to obtain the tracking result. Then use the scale filter for scale estimation. By setting the scale pool, train the...
Embodiment 3
[0222] The embodiment of the present invention also provides a twin network video tracking device based on a layered attention mechanism, including a processor and a storage medium;
[0223] The storage medium is used to store instructions;
[0224] The processor is configured to operate according to the instructions to execute the steps of the method in the first embodiment.
PUM
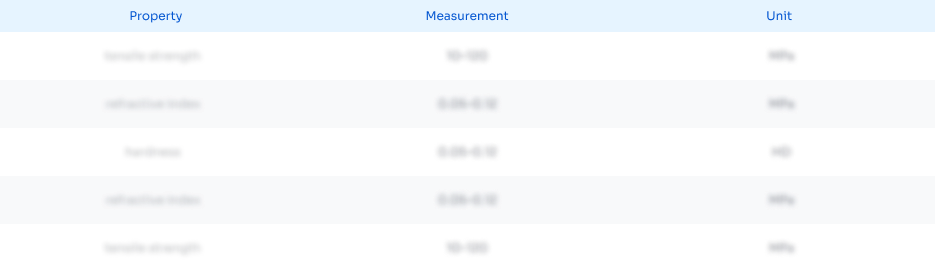
Abstract
Description
Claims
Application Information
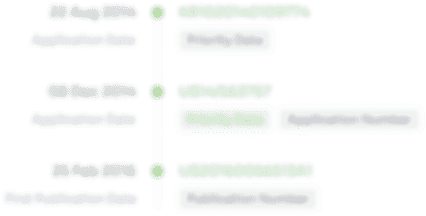
- R&D
- Intellectual Property
- Life Sciences
- Materials
- Tech Scout
- Unparalleled Data Quality
- Higher Quality Content
- 60% Fewer Hallucinations
Browse by: Latest US Patents, China's latest patents, Technical Efficacy Thesaurus, Application Domain, Technology Topic, Popular Technical Reports.
© 2025 PatSnap. All rights reserved.Legal|Privacy policy|Modern Slavery Act Transparency Statement|Sitemap|About US| Contact US: help@patsnap.com