Deep learning image registration method and device, electronic equipment and storage medium
A technology of deep learning and electronic equipment, applied in the field of image processing, can solve problems such as slow registration speed
- Summary
- Abstract
- Description
- Claims
- Application Information
AI Technical Summary
Problems solved by technology
Method used
Image
Examples
Embodiment 1
[0043] figure 1 It is a schematic flowchart of a deep learning image registration method provided by an embodiment of the present invention. The deep learning image registration method provided by this embodiment is applicable to a deep neural network, and the method can be executed by an electronic device. Specifically Specifically, the registration method of a deep learning image provided in this embodiment includes the following steps:
[0044] Step 100, acquire the deformation fields of the first fixed image and the moving image through a first algorithm.
[0045]In this embodiment, this embodiment is mainly applicable to the field of nuclear magnetic resonance imaging, specifically, nuclear magnetic resonance imaging (Nuclear Magnetic Resonance Imaging, NMRI for short), also known as spin imaging (spin imaging), also known as magnetic resonance imaging (Magnetic resonance imaging). Resonance Imaging, referred to as MRI), is to use the principle of nuclear magnetic resona...
Embodiment 2
[0060] figure 2 It is a method flowchart of a deep learning image registration method provided in Embodiment 2 of the present invention. The deep learning image registration method provided in this embodiment is suitable for deep neural networks. This embodiment is based on Embodiment 1 Based on the expansion, specifically, the following steps are included:
[0061] Step 200, acquire the deformation fields of the first fixed image and the moving image through the first algorithm.
[0062] Step 210, acquire a second fixed image according to the deformation field and the moving image.
[0063] Step 220, taking the second fixed image and moving image as input.
[0064] Step 230 , using the deformation field as an output to train an untrained registration network to obtain a trained registration network.
[0065] In this embodiment, the deep neural network is a registration network, and an untrained registration network is pre-trained through various parameter sets to obtain a...
Embodiment 3
[0082] The deep learning image registration device of the embodiment of the present invention can implement the deep learning image registration method provided by any embodiment of the present invention, and has corresponding functional modules and beneficial effects for executing the method. image 3 It is a schematic structural diagram of a deep learning image registration device 300 in an embodiment of the present invention. refer to image 3 , the deep learning image registration device 300 provided by the embodiment of the present invention may specifically include:
[0083] An acquisition module 300, configured to acquire the deformation fields of the first fixed image and the moving image through a first algorithm;
[0084] A generating module 310, configured to acquire a second fixed image according to the deformation field and the moving image;
[0085]The output module 320 is configured to train the deep neural network according to the moving image, the second fix...
PUM
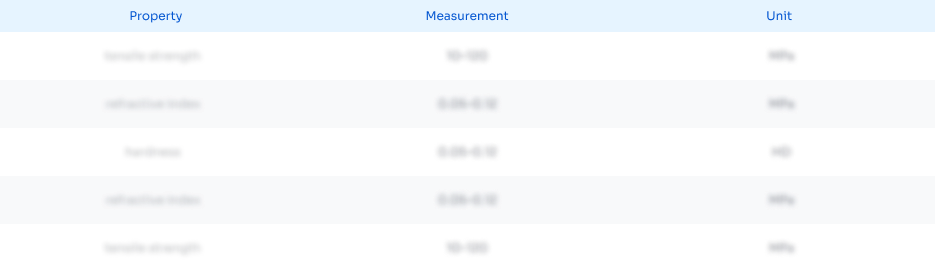
Abstract
Description
Claims
Application Information
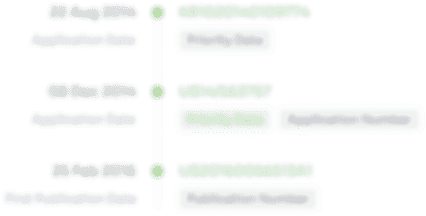
- R&D Engineer
- R&D Manager
- IP Professional
- Industry Leading Data Capabilities
- Powerful AI technology
- Patent DNA Extraction
Browse by: Latest US Patents, China's latest patents, Technical Efficacy Thesaurus, Application Domain, Technology Topic, Popular Technical Reports.
© 2024 PatSnap. All rights reserved.Legal|Privacy policy|Modern Slavery Act Transparency Statement|Sitemap|About US| Contact US: help@patsnap.com