Power load probability prediction method based on constrained parallel LSTM quantile regression
A quantile regression and power load technology, applied in forecasting, neural learning methods, data processing applications, etc., can solve unreasonable, crossover and other problems, achieve high forecasting efficiency, avoid crossover, and predict load probability distribution reasonably
- Summary
- Abstract
- Description
- Claims
- Application Information
AI Technical Summary
Problems solved by technology
Method used
Image
Examples
Embodiment Construction
[0039] Such as figure 1 As shown, the power load probability prediction method based on constrained parallel LSTM quantile regression includes the following steps. Step 1: Collect load data at intervals of 15 minutes from January 1, 2016 to June 30, 2017 in an actual area, Temperature data and rainfall, form a data set and divide it into training set, verification set and test set according to the ratio of 8:1:1, input variable X d =[T d , R d ], including the temperature T at 24 hours of the forecast day d =[T 1 , T 2 ,...,T 24 ] d and the rainfall R of the M divisions d =[R 1 , R 2 ,...,R M ] d ; Considering that the data difference between the data is relatively large, it is necessary to normalize different data into [-1,1], and the input sample after normalization processing is x' i; The sample data before normalization processing is x i , and its maximum and minimum sample values are respectively x , the number of samples is N, and the specific processi...
PUM
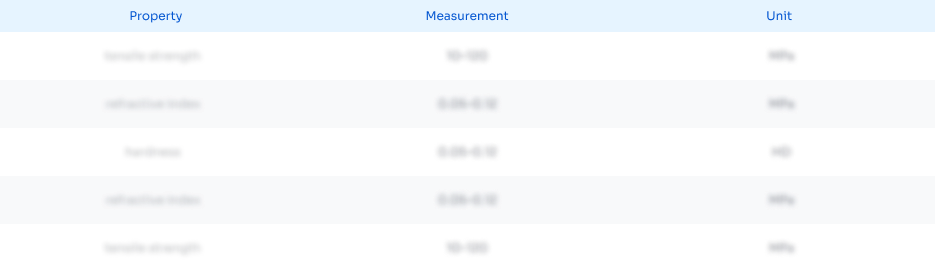
Abstract
Description
Claims
Application Information
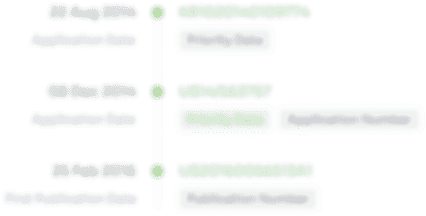
- R&D Engineer
- R&D Manager
- IP Professional
- Industry Leading Data Capabilities
- Powerful AI technology
- Patent DNA Extraction
Browse by: Latest US Patents, China's latest patents, Technical Efficacy Thesaurus, Application Domain, Technology Topic, Popular Technical Reports.
© 2024 PatSnap. All rights reserved.Legal|Privacy policy|Modern Slavery Act Transparency Statement|Sitemap|About US| Contact US: help@patsnap.com