Cascaded cavity convolutional network brain tumor segmentation method with attention mechanism
A convolutional network and attention technology, applied in the field of 3D medical brain tumor image segmentation, can solve problems such as error-prone, time-consuming and labor-intensive, and achieve the effect of reducing the number imbalance, reducing the complexity, and reducing the consumption of network parameters and video memory
- Summary
- Abstract
- Description
- Claims
- Application Information
AI Technical Summary
Problems solved by technology
Method used
Image
Examples
Embodiment Construction
[0034] Firstly, the technical scheme of the brain tumor segmentation of the cascaded hollow convolutional network with attention mechanism of the present invention is introduced, and the steps are as follows:
[0035] (1) Data preprocessing:
[0036] This invention uses the published BraTS 2018 [6] The data set includes 285 cases in the training set and 66 cases in the verification set. The training set contains 210 cases of HGG and 75 cases of LGG. Each case has 3D MR images of four modes: T1, T1ce, T2 and FLAIR, and each size is 240×240×155; the validation set has 66 cases and does not distinguish between tumor types. In the axial, sagittal and coronal directions, it is divided into 144×144×19 blocks as the original input.
[0037] (2) Network structure construction, the method is as follows:
[0038] The cascaded hole convolutional network with attention mechanism adopts a three-level cascade framework, which simplifies multi-class segmentation tasks into three second-cl...
PUM
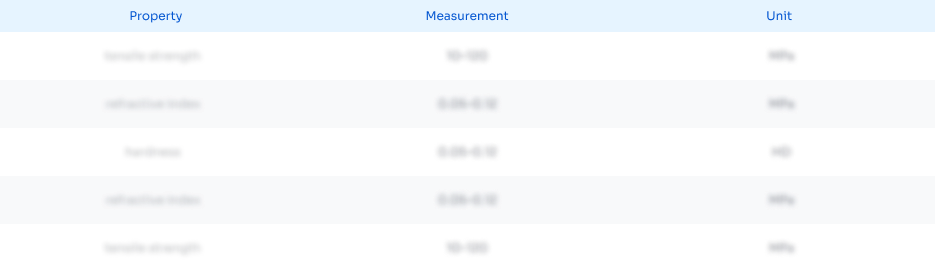
Abstract
Description
Claims
Application Information
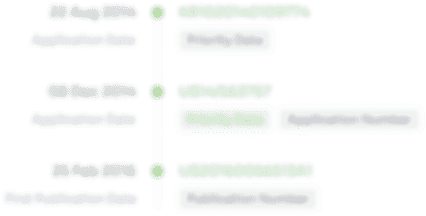
- R&D
- Intellectual Property
- Life Sciences
- Materials
- Tech Scout
- Unparalleled Data Quality
- Higher Quality Content
- 60% Fewer Hallucinations
Browse by: Latest US Patents, China's latest patents, Technical Efficacy Thesaurus, Application Domain, Technology Topic, Popular Technical Reports.
© 2025 PatSnap. All rights reserved.Legal|Privacy policy|Modern Slavery Act Transparency Statement|Sitemap|About US| Contact US: help@patsnap.com