Partial discharge mode recognition method of deep super learning machine in combination with evidence discount
A partial discharge and evidence discounting technology, which is applied in character and pattern recognition, pattern recognition in signals, machine learning, etc., can solve the problems of poor recognition effect, high-dimensional complexity, poor interpretability of results, etc., and achieve comprehensive and reliable recognition effect, low pattern recognition results, addressing effects with poor interpretability
- Summary
- Abstract
- Description
- Claims
- Application Information
AI Technical Summary
Problems solved by technology
Method used
Image
Examples
Embodiment
[0042] Such as figure 1 As shown, the partial discharge pattern recognition method of deep super learning machine combined with evidence discounting includes the following steps:
[0043] (S1) Signal acquisition. Collect partial discharge signals of electrical equipment through sensors, such as high-frequency sensors, ultra-high frequency sensors, etc.;
[0044] (S2) Data preprocessing. Due to factors such as the collection site environment, the original signal will contain various interferences, and methods such as wavelet analysis can be used to denoise the partial discharge signal to improve the accuracy of subsequent pattern recognition;
[0045] (S3) The deep super learning machine initially diagnoses the partial discharge signal, and constructs BPA of multiple evidence bodies. The processed partial discharge signal is used as input and sent to the deep super learning machine. The deep super learning machine in the present invention integrates five traditional machine...
PUM
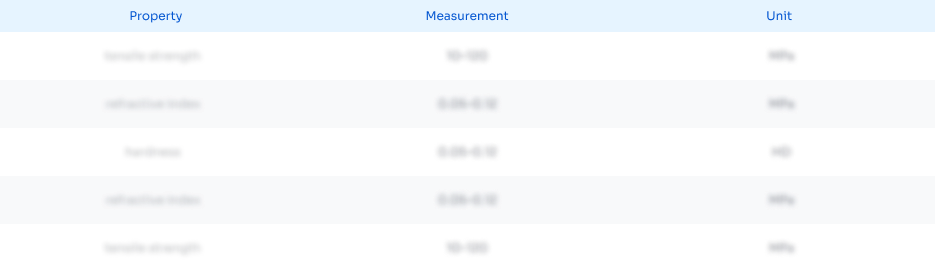
Abstract
Description
Claims
Application Information
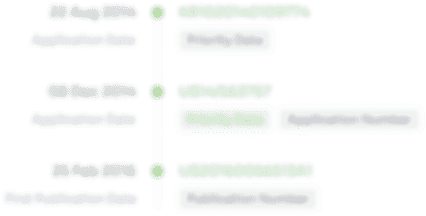
- Generate Ideas
- Intellectual Property
- Life Sciences
- Materials
- Tech Scout
- Unparalleled Data Quality
- Higher Quality Content
- 60% Fewer Hallucinations
Browse by: Latest US Patents, China's latest patents, Technical Efficacy Thesaurus, Application Domain, Technology Topic, Popular Technical Reports.
© 2025 PatSnap. All rights reserved.Legal|Privacy policy|Modern Slavery Act Transparency Statement|Sitemap|About US| Contact US: help@patsnap.com