Kidney tumor segmentation method based on multi-scale feature learning
A multi-scale feature, kidney tumor technology, applied in the field of medical image processing, can solve problems such as difficult detection results and false negative results, and achieve the effect of overcoming volume sensitivity, high accuracy, and improving performance
- Summary
- Abstract
- Description
- Claims
- Application Information
AI Technical Summary
Problems solved by technology
Method used
Image
Examples
Embodiment
[0032] Cooperate Figure 1 to Figure 4 As shown, the present invention discloses a renal tumor segmentation method based on multi-scale feature learning, comprising the following steps:
[0033] S1. Obtain an abdominal scan image, and divide the acquired abdominal scan image into a training set.
[0034] S2. Preprocessing the abdominal scan images in the training set to obtain preprocessed images.
[0035] S3. Construct a multi-scale feature network, and combine the pyramid pooling module and the feature pyramid fusion module through the network to capture the global structural information of the image to perform accurate kidney segmentation.
[0036] S4. Predict and segment the preprocessed image in S2 through a multi-scale feature network.
[0037] The preprocessing operation in step S2 is specifically to down-sample the acquired abdominal scan image by 4 mm at the sampling distance in the three directions of XYZ, and the zoomed image is 1 / 16 of the original size, and re-u...
PUM
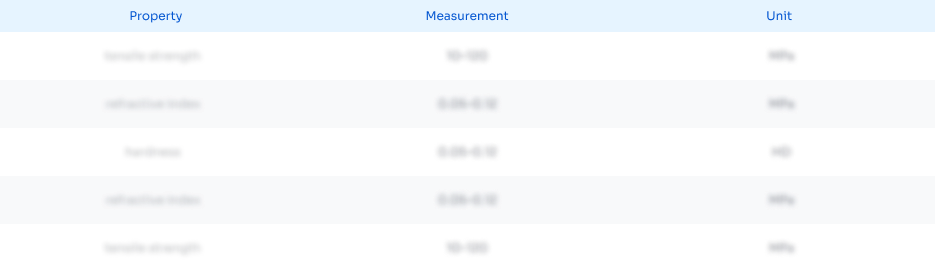
Abstract
Description
Claims
Application Information
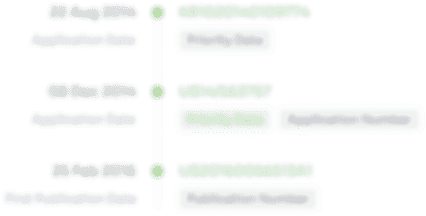
- R&D Engineer
- R&D Manager
- IP Professional
- Industry Leading Data Capabilities
- Powerful AI technology
- Patent DNA Extraction
Browse by: Latest US Patents, China's latest patents, Technical Efficacy Thesaurus, Application Domain, Technology Topic, Popular Technical Reports.
© 2024 PatSnap. All rights reserved.Legal|Privacy policy|Modern Slavery Act Transparency Statement|Sitemap|About US| Contact US: help@patsnap.com