Brushless direct current motor sensor fault detection method based on convolutional neural network
A technology of convolutional neural network and brushed DC motor, which is applied to biological neural network models, neural architectures, instruments, etc., can solve the problems of complex detection methods and limited application occasions, and achieve the goal of improving prediction accuracy and reducing failure rate Effect
- Summary
- Abstract
- Description
- Claims
- Application Information
AI Technical Summary
Problems solved by technology
Method used
Image
Examples
Embodiment Construction
[0020] In order to make the object, technical solution and advantages of the present invention clearer, the present invention will be further described in detail below in conjunction with the accompanying drawings.
[0021] Specific embodiments of the present invention such as figure 1 figure 2 Shown is the system block diagram of the brushless DC motor system. The brushless DC motor system is mainly composed of the motor body, the electronic commutation circuit and the rotor position sensor. The electronic commutation circuit mainly includes two parts, which are divided into: drive and control parts. In the control system, the high-precision control board is the control core, which processes, calculates and analyzes Hall sensor signals, counter electromotive force zero-crossing signals, voltage signals, current signals, etc., and outputs corresponding signals at the same time to realize the inverter On and off, and then realize effective control of the operation of the bru...
PUM
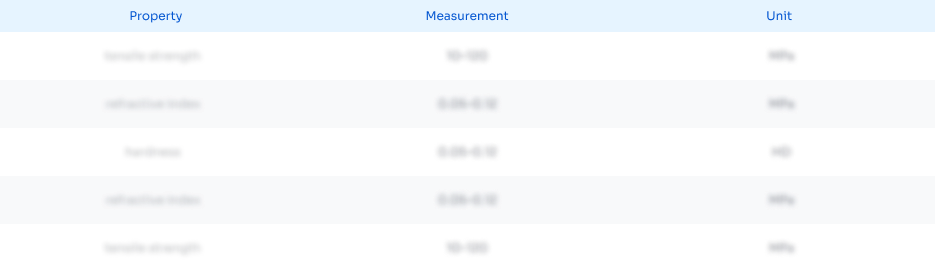
Abstract
Description
Claims
Application Information
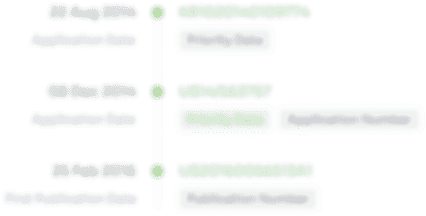
- R&D Engineer
- R&D Manager
- IP Professional
- Industry Leading Data Capabilities
- Powerful AI technology
- Patent DNA Extraction
Browse by: Latest US Patents, China's latest patents, Technical Efficacy Thesaurus, Application Domain, Technology Topic, Popular Technical Reports.
© 2024 PatSnap. All rights reserved.Legal|Privacy policy|Modern Slavery Act Transparency Statement|Sitemap|About US| Contact US: help@patsnap.com