Well-to-seismic joint initial lithologic model construction method based on deep learning
A well-seismic combination and deep learning technology, applied in neural learning methods, biological neural network models, seismology, etc., can solve problems such as low logging data frequency, gradient disappearance, and insufficient initial model resolution, and achieve high computational efficiency , reliable results, fast convergence
- Summary
- Abstract
- Description
- Claims
- Application Information
AI Technical Summary
Problems solved by technology
Method used
Image
Examples
Embodiment Construction
[0055] The accuracy of traditional initial lithology model construction directly affects the inversion of lithology parameters, thus determining the final lithology model. At present, the commonly used initial lithology model construction method only uses seismic data, which leads to the lack of high-frequency information and insufficient resolution of the initial model; it does not make full use of logging data, and the information mining of seismic data and logging data is limited, resulting in incomplete initial models. precise. The present invention is based on deep learning, and proposes a new neural network, which uses the convolutional neural network to extract the long and short cycle, that is, the high and low frequency features contained in the data, and uses the long and short time memory network to perform feature selection and classification for different features. Furthermore, the relationship between seismic data and well logging data can be learned accurately t...
PUM
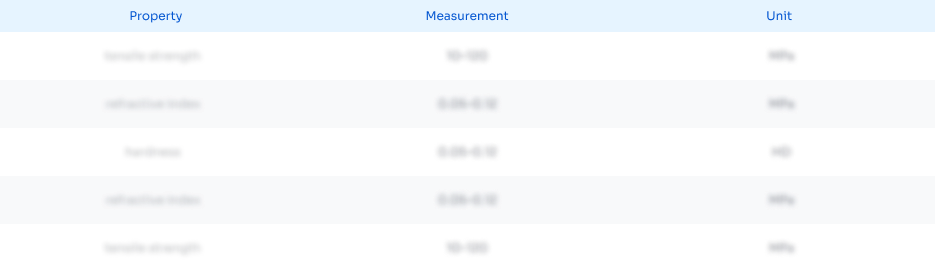
Abstract
Description
Claims
Application Information
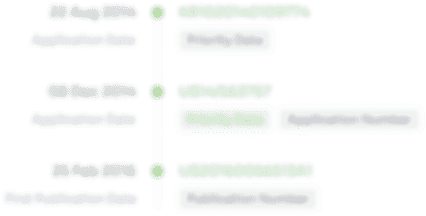
- R&D Engineer
- R&D Manager
- IP Professional
- Industry Leading Data Capabilities
- Powerful AI technology
- Patent DNA Extraction
Browse by: Latest US Patents, China's latest patents, Technical Efficacy Thesaurus, Application Domain, Technology Topic, Popular Technical Reports.
© 2024 PatSnap. All rights reserved.Legal|Privacy policy|Modern Slavery Act Transparency Statement|Sitemap|About US| Contact US: help@patsnap.com