Unmanned aerial vehicle network hovering position optimization method based on multi-agent deep reinforcement learning
A reinforcement learning, multi-agent technology, applied in neural learning methods, network planning, biological neural network models, etc., can solve the problems of UAVs trajectory optimization method not applicable to the actual communication environment, ignoring service fairness, centralized control difficulties, etc.
- Summary
- Abstract
- Description
- Claims
- Application Information
AI Technical Summary
Problems solved by technology
Method used
Image
Examples
Embodiment Construction
[0049] In order to make the object, technical solution and advantages of the present invention more clear, the present invention will be further described in detail below in conjunction with the examples. It should be understood that the specific embodiments described here are only used to explain the present invention, not to limit the present invention.
[0050] A multi-agent deep reinforcement learning based hovering position optimization method for UAV networks, applied to emergency communication restoration in areas lacking ground infrastructure or after disasters. Such as figure 1 As shown, the area lacks basic communication facilities, and UAVs are used as mobile base stations for communication coverage. The ground environment is dynamically changing, and ground equipment may move. The UAV base station needs to constantly adjust its hovering position to achieve Better communication services (maximize system throughput). At the same time, service fairness and energy co...
PUM
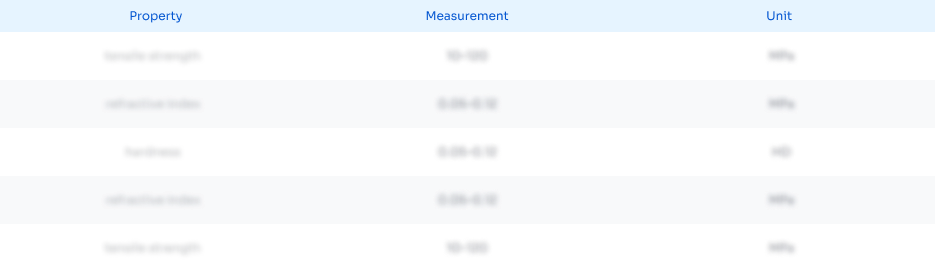
Abstract
Description
Claims
Application Information
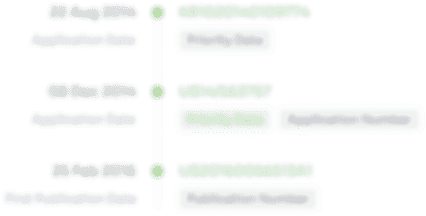
- R&D Engineer
- R&D Manager
- IP Professional
- Industry Leading Data Capabilities
- Powerful AI technology
- Patent DNA Extraction
Browse by: Latest US Patents, China's latest patents, Technical Efficacy Thesaurus, Application Domain, Technology Topic, Popular Technical Reports.
© 2024 PatSnap. All rights reserved.Legal|Privacy policy|Modern Slavery Act Transparency Statement|Sitemap|About US| Contact US: help@patsnap.com