Computer classification polygraph recognition method based on fmri small world brain network
A recognition method and brain network technology, applied in the field of computer classification and polygraph recognition based on fMRI small-world brain network, achieves the effect of low calculation cost and good generalization performance
- Summary
- Abstract
- Description
- Claims
- Application Information
AI Technical Summary
Problems solved by technology
Method used
Image
Examples
Embodiment 1
[0055] Embodiment 1: see figure 1 , a kind of computer classification polygraph recognition method based on fMRI small-world brain network, comprises the following steps:
[0056] (1) Obtain the functional magnetic resonance image data of the subject;
[0057] (11) Select N subjects, among which N1 subjects are used as training samples, and N2 subjects are used as test samples, and N=N1+N2, N1>>N2;
[0058] (12) Collect the fMRI images of N1 training samples under the lying and honest cognitive experiments, and perform preprocessing to obtain the preprocessed fMRI images, and the fMRI images are accompanied by labels corresponding to lying and honesty category;
[0059] (13) For N2 samples to be tested, collect fMRI images of their brains, and perform preprocessing to obtain preprocessed fMRI images;
[0060] (2) Construct the subject's binary matrix;
[0061] For each subject, the preprocessed fMRI images were mapped to the 90 brain regions of the anatomically automatical...
Embodiment 2
[0074] Example 2, see figure 1 , this embodiment is further supplemented on the basis of embodiment 1.
[0075] In the step (1), the preprocessing is: perform temporal layer correction; perform head movement correction to remove motion artifacts; standardize the functional image to the EPI template that comes with SPM, and use the half-maximum width FWHM of 8mm to perform spatial smoothing on the data , and finally use a high-pass filter with a cutoff frequency of 1 / 128Hz to filter out the low-frequency noise of the signal.
[0076] The step (2) threshold range meets the following conditions:
[0077] First, call the corrcoef function in MATLAB to complete the calculation of the Pearson correlation coefficient. The minimum value of the threshold range can keep the corresponding Pearson correlation coefficient calculated by the corrcoef function with a significant p<0.05, and exclude the corresponding ones that are not significant Pearson correlation coefficient;
[0078] Se...
Embodiment 3
[0092] Embodiment 3: see figure 1 , Fig. 2, in order to verify the classification accuracy rate of a computerized polygraph recognition method based on fMRI small-world brain network mentioned in the present invention, we analyzed the fMRI images of 36 subjects with known category labels and The method was tested using leave-one-out cross-validation. The leave-one-out approach is based on a single measurement technique architecture (see figure 1 ) as the core but involves multiple measurements in a cycle, the description of the operation process is as follows:
[0093] (1) Preprocess the fMRI images of the subject: perform time layer correction; perform head movement correction to remove motion artifacts; normalize the functional image to the EPI template that comes with SPM, and use 8mm half-maximum width FWHM to process the data Spatial smoothing, and finally a high-pass filter with a cutoff frequency of 1 / 128Hz is used to filter out the low-frequency noise of the signal t...
PUM
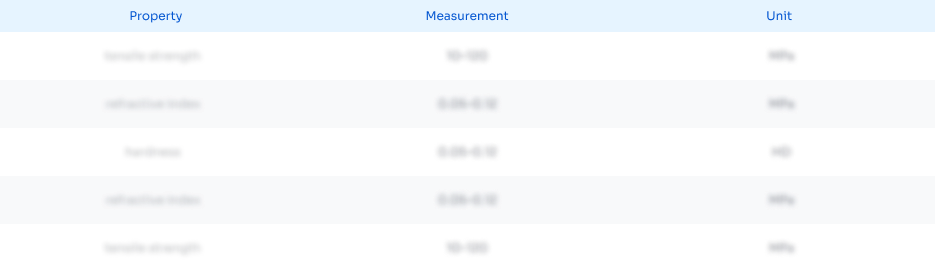
Abstract
Description
Claims
Application Information
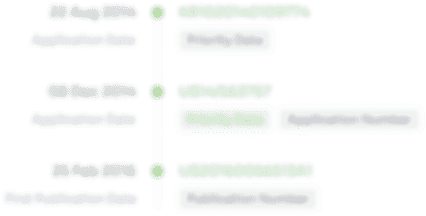
- R&D Engineer
- R&D Manager
- IP Professional
- Industry Leading Data Capabilities
- Powerful AI technology
- Patent DNA Extraction
Browse by: Latest US Patents, China's latest patents, Technical Efficacy Thesaurus, Application Domain, Technology Topic, Popular Technical Reports.
© 2024 PatSnap. All rights reserved.Legal|Privacy policy|Modern Slavery Act Transparency Statement|Sitemap|About US| Contact US: help@patsnap.com