Super-large image classification method based on graph neural network
A neural network and classification method technology, applied in the direction of instruments, scene recognition, computing, etc., can solve problems such as complex classification tasks, complex label data, computer memory overflow, etc.
- Summary
- Abstract
- Description
- Claims
- Application Information
AI Technical Summary
Problems solved by technology
Method used
Image
Examples
Embodiment Construction
[0072] The specific implementation manners of the present invention will be further described below in conjunction with the accompanying drawings and technical solutions.
[0073] The present invention can be used for the classification task of multiple super-large images, and the process of the present invention is as follows figure 1 As shown, the graph network structure adopted is as follows figure 2 shown.
[0074] This embodiment is applied to the classification task of medical pathological images and remote sensing images, and the specific embodiments discussed are only used to illustrate the implementation of the present invention, and do not limit the scope of the present invention.
[0075] The embodiment of the present invention will be described in detail below mainly aiming at the problem of medical pathological images, specifically including the following steps (such as image 3 shown):
[0076] (1) Perform data and processing according to module 1 in the cont...
PUM
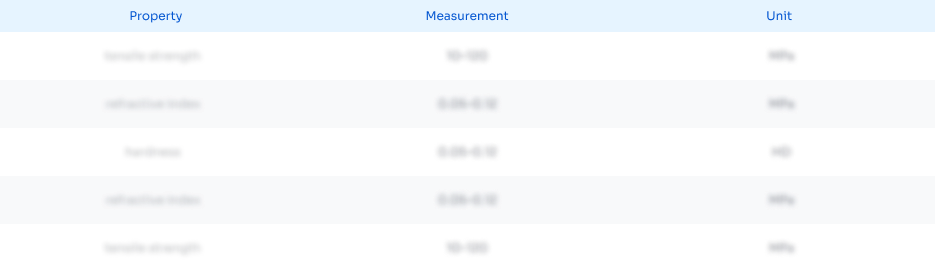
Abstract
Description
Claims
Application Information
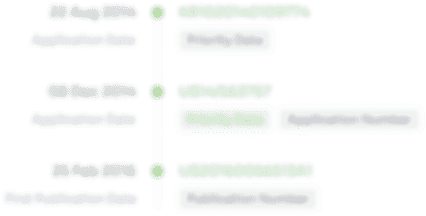
- R&D
- Intellectual Property
- Life Sciences
- Materials
- Tech Scout
- Unparalleled Data Quality
- Higher Quality Content
- 60% Fewer Hallucinations
Browse by: Latest US Patents, China's latest patents, Technical Efficacy Thesaurus, Application Domain, Technology Topic, Popular Technical Reports.
© 2025 PatSnap. All rights reserved.Legal|Privacy policy|Modern Slavery Act Transparency Statement|Sitemap|About US| Contact US: help@patsnap.com