Joint neural network model compression method based on channel pruning and quantitative training
A compression method and technology for training models, which are applied in the field of joint neural network model compression, and can solve the problems of large number of parameters of neural network models, difficult to deploy, and large amount of calculation.
- Summary
- Abstract
- Description
- Claims
- Application Information
AI Technical Summary
Problems solved by technology
Method used
Image
Examples
Embodiment 1
[0088] A joint neural network model compression method based on channel pruning and quantization training, the compression method comprises the following steps: channel pruning is to reduce the number of neural network channels; quantization training replaces floating-point number operations with integer operations
[0089] Step 1: Sparse the training model. During the training process, apply the L1 norm penalty to the BN layer parameters after the convolutional layer that needs to be sparse, so that the parameters have the characteristics of structured sparseness, and prepare for the next step of cutting the channel;
[0090] Step 2: Training model pruning. According to the corresponding relationship between the convolutional layer and the BN layer in the model, the pruning process cuts off the channel corresponding to the convolutional layer with a small γ parameter in the BN layer, and prunes each layer from shallow to deep. , thus forming a new model after channel pruning; ...
Embodiment 2
[0158] The improved YOLOv3 network is compressed using the pruning algorithm of the present invention. The improved YOLOv3 network structure uses Mobilenetv2 as the feature extractor, and then replaces the ordinary convolution with a depth-separable convolution to reduce the amount of calculation. The improved YOLOv3 network achieves a test set mAP of 78.46% on the VOC dataset. On a 512×512 input image, the calculation amount is 4.15GMACs, and the model parameter amount is 6.775M.
[0159] The above results were trained for 80 rounds on the VOC training set, using standard data enhancement methods, including random cropping, perspective transformation, and horizontal flip, and additionally using the mixup data enhancement method. Using the Adam optimization algorithm, the learning rate strategy of cosine annealing, the initial learning rate is 4e-3, and the batch size is 16. The following sparse training and fine-tuning training use the same hyperparameter settings.
[0160...
PUM
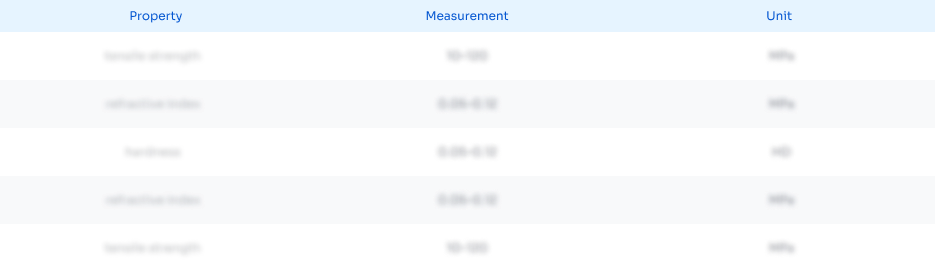
Abstract
Description
Claims
Application Information
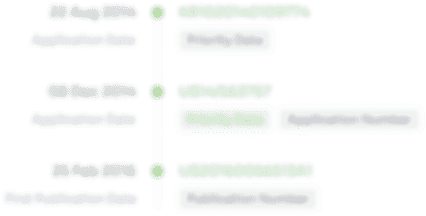
- R&D Engineer
- R&D Manager
- IP Professional
- Industry Leading Data Capabilities
- Powerful AI technology
- Patent DNA Extraction
Browse by: Latest US Patents, China's latest patents, Technical Efficacy Thesaurus, Application Domain, Technology Topic.
© 2024 PatSnap. All rights reserved.Legal|Privacy policy|Modern Slavery Act Transparency Statement|Sitemap