Method for constructing human body behavior recognition model based on graph convolution network
A convolutional network and recognition model technology, applied in the field of human behavior recognition model construction, can solve problems such as fixed graph structure is not optimal, ST-GCN does not support dependencies, etc., to achieve the effect of improving recognition performance
- Summary
- Abstract
- Description
- Claims
- Application Information
AI Technical Summary
Problems solved by technology
Method used
Image
Examples
Embodiment Construction
[0065] like figure 1 As shown, a method for constructing a human behavior recognition model based on a graph convolutional network of the present invention includes the following steps:
[0066] Step 1, skeleton sequence acquisition and preprocessing;
[0067] Step 2, constructing a spatiotemporal graph representing the skeleton sequence;
[0068] Step 3, constructing a three-stream graph convolutional network based on the spatiotemporal graph; the three-stream graph convolutional network includes three graphs for modeling three kinds of information of joint points, skeletons and skeletal motions on the input spatiotemporal graph respectively. Convolutional network, the three graph convolutional networks are the same, and the output of each graph convolutional network is fused as the output of the three-stream graph convolutional network;
[0069] Step 4: After converting the skeleton sequence obtained in step 1 into a spatiotemporal graph in step 2, input the three-stream ...
PUM
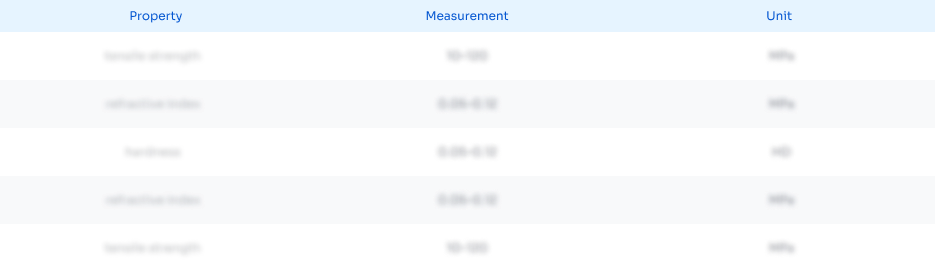
Abstract
Description
Claims
Application Information
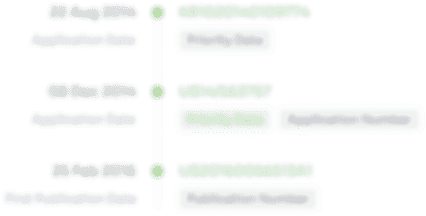
- R&D Engineer
- R&D Manager
- IP Professional
- Industry Leading Data Capabilities
- Powerful AI technology
- Patent DNA Extraction
Browse by: Latest US Patents, China's latest patents, Technical Efficacy Thesaurus, Application Domain, Technology Topic, Popular Technical Reports.
© 2024 PatSnap. All rights reserved.Legal|Privacy policy|Modern Slavery Act Transparency Statement|Sitemap|About US| Contact US: help@patsnap.com