Machine fault prediction and diagnosis method based on three-level neural network modeling
A neural network modeling and machine failure technology, applied in biological neural network models, neural learning methods, neural architectures, etc., can solve problems such as long-term data accumulation and limited predictive diagnosis
- Summary
- Abstract
- Description
- Claims
- Application Information
AI Technical Summary
Problems solved by technology
Method used
Image
Examples
Embodiment Construction
[0089] The machine failure prediction and diagnosis method based on three-level neural network modeling proposed by the present invention comprises the following steps:
[0090] (1) Obtain the running state monitoring data of the machine to be tested from the machine fault labeling log of the machine operation and maintenance management department. The running state monitoring data includes the speed data R, temperature data T, vibration data V and sound data S of the machine to be tested, R, T, V and S are time series data;
[0091] (2) Perform frame processing on the running status monitoring data collected in step (1), set the duration of the data frame as tlen, and start time of the i-th data frame as t i , intercept time window [t i ,t i R, T, V, S in +tlen] are recorded as R_t respectively i , T_t i , V_t i and S_t i , after frame processing, the R, T, V, and S data are all divided into N data frames, recorded as:
[0092]
[0093] Among them, N is the total nu...
PUM
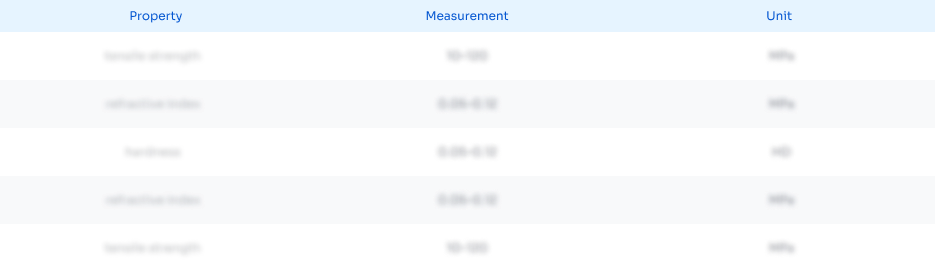
Abstract
Description
Claims
Application Information
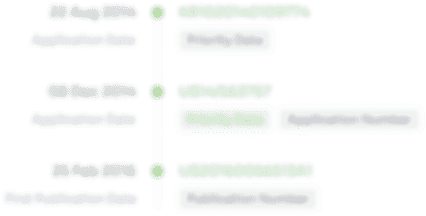
- R&D Engineer
- R&D Manager
- IP Professional
- Industry Leading Data Capabilities
- Powerful AI technology
- Patent DNA Extraction
Browse by: Latest US Patents, China's latest patents, Technical Efficacy Thesaurus, Application Domain, Technology Topic, Popular Technical Reports.
© 2024 PatSnap. All rights reserved.Legal|Privacy policy|Modern Slavery Act Transparency Statement|Sitemap|About US| Contact US: help@patsnap.com