Bearing fault diagnosis method based on transfer learning
A technology of transfer learning and fault diagnosis, which is applied in the direction of mechanical bearing testing, etc.
- Summary
- Abstract
- Description
- Claims
- Application Information
AI Technical Summary
Problems solved by technology
Method used
Image
Examples
Embodiment Construction
[0058] The present invention will be described in detail below in conjunction with the accompanying drawings and specific embodiments.
[0059] see figure 1 , a bearing fault diagnosis method based on transfer learning of the present invention comprises the following steps:
[0060] Step A: Use autocorrelation singular value decomposition (SVD) for the target data and auxiliary data to extract the contained fault singular value vectors (ie, fault feature vectors), and perform sample collection according to the selection rules of the training data set T and the test data set S select;
[0061] In step A, the target data source is: the vibration data of the target bearing object in the target environment, and the auxiliary data source is: the vibration data of the non-target environment or non-target object; the target data and auxiliary data of the bearing system are vibration acceleration signals, and the bearing An example of a vibration signal is shown in figure 2 shown;...
PUM
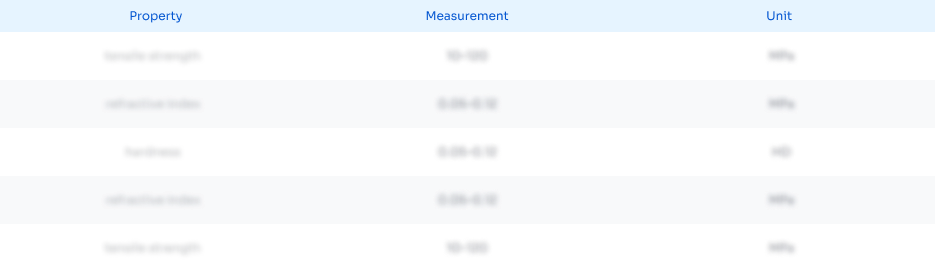
Abstract
Description
Claims
Application Information
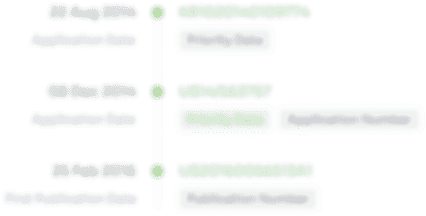
- R&D Engineer
- R&D Manager
- IP Professional
- Industry Leading Data Capabilities
- Powerful AI technology
- Patent DNA Extraction
Browse by: Latest US Patents, China's latest patents, Technical Efficacy Thesaurus, Application Domain, Technology Topic, Popular Technical Reports.
© 2024 PatSnap. All rights reserved.Legal|Privacy policy|Modern Slavery Act Transparency Statement|Sitemap|About US| Contact US: help@patsnap.com