One-way valve fault feature extraction method based on improved permutation entropy
A technology of fault features and extraction methods, which is used in mechanical valve testing, pattern recognition in signals, complex mathematical operations, etc. It can solve problems such as loss of information and affecting diagnostic efficiency.
- Summary
- Abstract
- Description
- Claims
- Application Information
AI Technical Summary
Problems solved by technology
Method used
Image
Examples
Embodiment 1
[0056] Embodiment 1: as figure 1 As shown, a check valve fault feature extraction method based on improved permutation entropy, the specific steps are:
[0057] Step1: Use the acceleration sensor to sample when the check valve is normal, the valve is stuck, and the wear is faulty, and the vibration data of the check valve is obtained in each state;
[0058] Step2: Improve the permutation entropy by adding a weighted coefficient to the reconstruction component of the permutation entropy, and propose an improved permutation entropy algorithm;
[0059] Step3: Use the improved permutation entropy to directly extract the fault features of the check valve under the background of strong noise, and realize the fault diagnosis of the check valve.
[0060] The specific steps of the permutation entropy algorithm in the Step2 are:
[0061] Step2.1: The discrete time series of length T Perform phase space reconstruction to obtain the reconstruction matrix Where m is the embedding di...
Embodiment 2
[0075] Embodiment 2: The present invention uses simulation signals to verify the effectiveness and superiority of improving permutation entropy from the aspects of detecting signal mutations, measuring signal complexity and anti-noise ability.
[0076] First, use the simulation signal 1 to verify the advantages of the improved permutation entropy in detecting signal mutations. The simulation signals are Gaussian white noise signals and shock signals, such as image 3 shown. In the figure, the amplitude of the shock signal is 50, the mean of Gaussian white noise is 0, and the standard deviation is 1. Calculate the PE and IPE of the simulated signal 1, Figure 4 It is the average value of 50 calculation results for the simulated signal. In the calculation process, a sliding window is constructed with 100 data points, and the sliding step is 10.
[0077] from Figure 4 It can be seen from the figure that when the sliding window only contains Gaussian white noise, the randomne...
Embodiment 3
[0082] Embodiment 3: The experimental data of this example comes from the rolling bearing data of the electrical engineering laboratory of Case Western Reserve University in the United States, select 25 groups of data of rolling bearing normal state, inner ring fault, outer ring fault, and rolling element fault, and the length of the data for 2400. Because the data is the data of laboratory simulated faults, the data is almost free of noise and relatively regular. Using improved permutation entropy to directly extract fault features, the experimental results are as follows Figure 8 shown.
[0083] Depend on Figure 8 It can be seen that since the data of rolling bearings contains almost no noise, the fault characteristics of bearings can be extracted effectively by directly using the improved permutation entropy. The IPE in the normal state is between 0.5-0.6, the outer ring fault is between 0.6-0.7, the rolling element fault is between 0.7-0.8, the inner ring fault is bet...
PUM
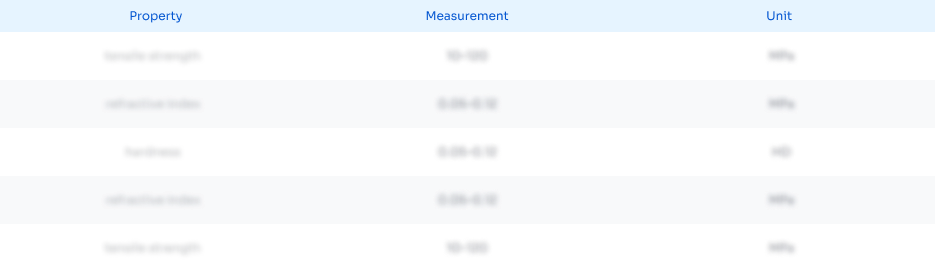
Abstract
Description
Claims
Application Information
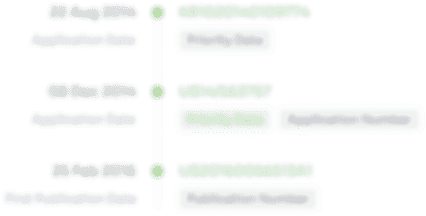
- R&D Engineer
- R&D Manager
- IP Professional
- Industry Leading Data Capabilities
- Powerful AI technology
- Patent DNA Extraction
Browse by: Latest US Patents, China's latest patents, Technical Efficacy Thesaurus, Application Domain, Technology Topic, Popular Technical Reports.
© 2024 PatSnap. All rights reserved.Legal|Privacy policy|Modern Slavery Act Transparency Statement|Sitemap|About US| Contact US: help@patsnap.com