Turbulence degradation image semantic segmentation method based on boundary perception and adversarial learning
A degenerate image and semantic segmentation technology, applied in neural learning methods, character and pattern recognition, instruments, etc., can solve the problems of difficult to express texture information, difficult semantic segmentation, lack of data sets, etc., to improve high-level inconsistency, improve Semantic segmentation effect, improve the effect of rough segmentation results
- Summary
- Abstract
- Description
- Claims
- Application Information
AI Technical Summary
Problems solved by technology
Method used
Image
Examples
Embodiment Construction
[0048] The technical solutions in the embodiments of the present invention will be clearly and completely described below in conjunction with the accompanying drawings in the embodiments of the present invention. Obviously, the described embodiments are only part of the embodiments of the present invention, not all of them. Based on the embodiments of the present invention, all other embodiments obtained by persons of ordinary skill in the art without making creative efforts belong to the protection scope of the present invention.
[0049] Such as figure 1 Shown, the concrete realization steps of the present invention are as follows:
[0050] Step 1. Combining the turbulent physical imaging model with image processing algorithms (ie, image interpolation and image convolution methods). Using the atmospheric turbulence physical imaging model, the turbulence degradation simulation of the image is carried out, and the semantic segmentation dataset of the turbulence degradation i...
PUM
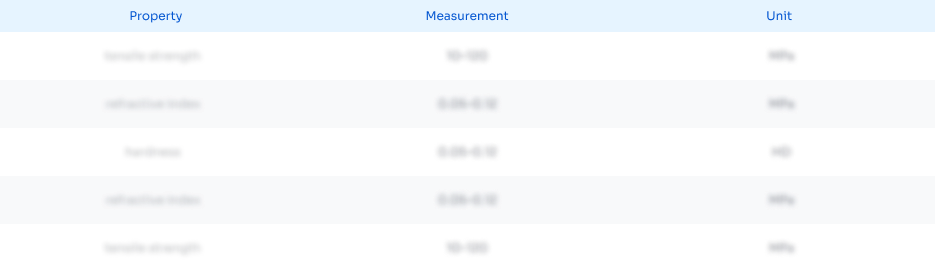
Abstract
Description
Claims
Application Information
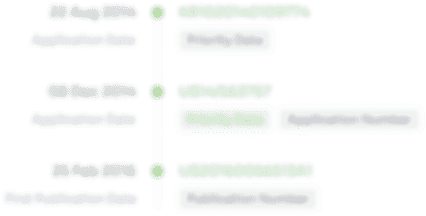
- R&D Engineer
- R&D Manager
- IP Professional
- Industry Leading Data Capabilities
- Powerful AI technology
- Patent DNA Extraction
Browse by: Latest US Patents, China's latest patents, Technical Efficacy Thesaurus, Application Domain, Technology Topic, Popular Technical Reports.
© 2024 PatSnap. All rights reserved.Legal|Privacy policy|Modern Slavery Act Transparency Statement|Sitemap|About US| Contact US: help@patsnap.com