A method for predicting the health status of lithium-ion batteries in electric forklifts based on lstm-ffnn
A lithium-ion battery, health status technology, applied in the direction of measuring electricity, measuring devices, measuring electrical variables, etc., can solve problems such as safety problems, economic losses, capacity attenuation, etc., to avoid economic losses and safety problems, stabilize energy supply, The effect of improving accuracy
- Summary
- Abstract
- Description
- Claims
- Application Information
AI Technical Summary
Problems solved by technology
Method used
Image
Examples
Embodiment
[0062] Embodiment: A LSTM-FFNN-based method for predicting the state of health of an electric forklift lithium-ion battery in this embodiment, the flow chart is as follows figure 1 As shown, the specific steps are as follows:
[0063] S1: Establish a time-scale parameter prediction model based on LSTM to predict the change of the time-scale parameter open circuit voltage V during the discharge process, including the following steps:
[0064] S11: Set the number of neurons in the input layer of the neural network as L1, set the number of hidden layers of the neural network N1 and the number of neurons L2, and complete the process from the input space U M to the output space U T The mapping of , that is, predict the open circuit voltage at the subsequent time according to the open circuit voltage at the first m moments;
[0065] S12: Set the moving step size as l, decompose and reconstruct the time scale parameter open circuit voltage data, and establish the training sample V ...
PUM
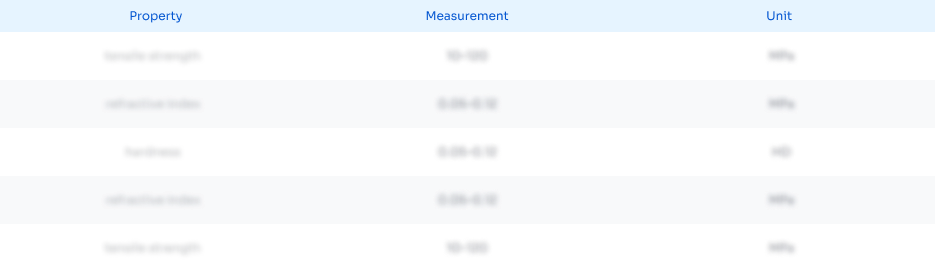
Abstract
Description
Claims
Application Information
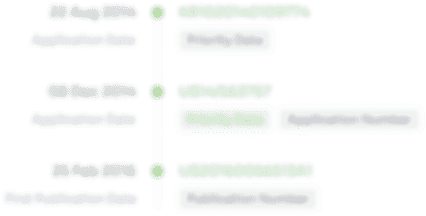
- Generate Ideas
- Intellectual Property
- Life Sciences
- Materials
- Tech Scout
- Unparalleled Data Quality
- Higher Quality Content
- 60% Fewer Hallucinations
Browse by: Latest US Patents, China's latest patents, Technical Efficacy Thesaurus, Application Domain, Technology Topic, Popular Technical Reports.
© 2025 PatSnap. All rights reserved.Legal|Privacy policy|Modern Slavery Act Transparency Statement|Sitemap|About US| Contact US: help@patsnap.com