Multi-label multi-mode holographic pulse condition recognition method based on graph convolution network
A convolutional network and multi-label technology, applied in the field of multi-label multi-modal holographic pulse recognition, can solve problems affecting efficiency, unable to represent lumen volume, blood flow velocity, vascular three-dimensional movement, and affecting model accuracy. The effect of improving efficiency
- Summary
- Abstract
- Description
- Claims
- Application Information
AI Technical Summary
Problems solved by technology
Method used
Image
Examples
Embodiment Construction
[0031] The present invention will be further described below in conjunction with the accompanying drawings. It should be noted that this embodiment is based on the technical solution, and provides detailed implementation and specific operation process, but the protection scope of the present invention is not limited to the present invention. Example.
[0032] This embodiment provides a multi-label multi-modal holographic pulse recognition method based on graph convolutional network, comprising the following steps:
[0033] S1. Based on the graph convolutional network, the relationship between label data and non-image features is mined.
[0034] Graph Convolutional Network (Graph Convolutional Network) is a method that can perform deep learning on graph data. The traditional convolutional neural network research object is still limited to the data of Euclidean domains. The most notable feature of Euclidean data is its regular spatial structure. For example, pictures are regul...
PUM
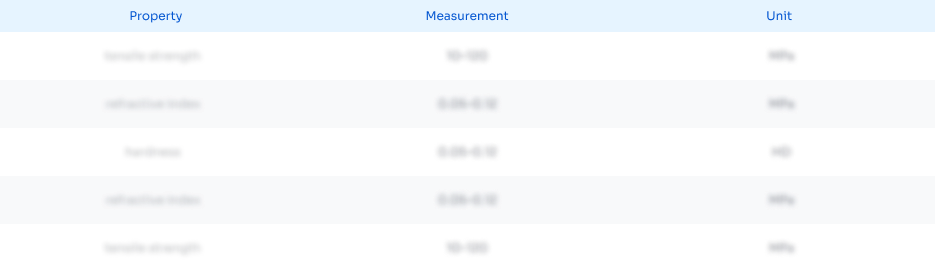
Abstract
Description
Claims
Application Information
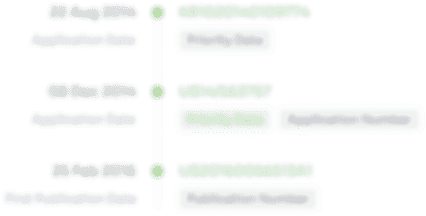
- R&D Engineer
- R&D Manager
- IP Professional
- Industry Leading Data Capabilities
- Powerful AI technology
- Patent DNA Extraction
Browse by: Latest US Patents, China's latest patents, Technical Efficacy Thesaurus, Application Domain, Technology Topic, Popular Technical Reports.
© 2024 PatSnap. All rights reserved.Legal|Privacy policy|Modern Slavery Act Transparency Statement|Sitemap|About US| Contact US: help@patsnap.com