Hyper-parameter asynchronous parallel search method based on population evolution
A search method and hyperparameter technology, applied in the field of hyperparameter asynchronous parallel search based on population evolution, can solve problems such as difficult to find, long hyperparameter search time, etc., and achieve the effect of performance improvement, time reduction and computational complexity
- Summary
- Abstract
- Description
- Claims
- Application Information
AI Technical Summary
Problems solved by technology
Method used
Image
Examples
Embodiment Construction
[0036] 1. PEHS algorithm construction process
[0037] The most common formulation in machine learning is to optimize the parameters θ of a model f to maximize a given objective function Q * (e.g. classification, reconstruction or prediction). The trainable parameters θ are typically updated by optimization procedures such as stochastic gradient descent. However, deep learning and reinforcement learning focus on the actual performance index Q, which is different from Q *Different (Jaderberg M, Dalibard V, Osindero S, et al. Population Based Training of Neural Networks. arXiv:1711.098462017.). For example, Q might be validation set accuracy, or environmental rewards in reinforcement learning). The main purpose of PEHS is to provide a method to simultaneously optimize the parameter θ and the hyperparameter h on the actual index Q.
[0038] First define an evaluation function eval() that uses the current state of the model to evaluate the objective function. For simplicity, ...
PUM
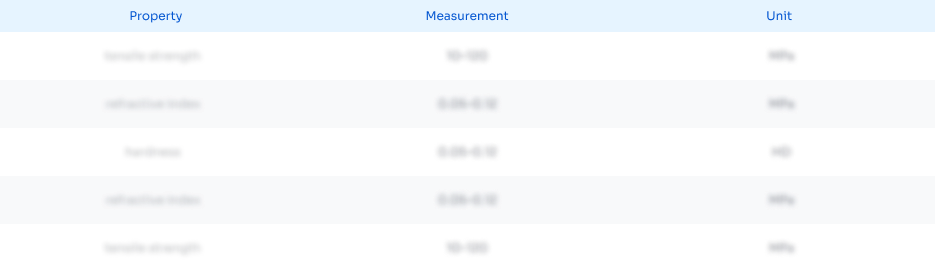
Abstract
Description
Claims
Application Information
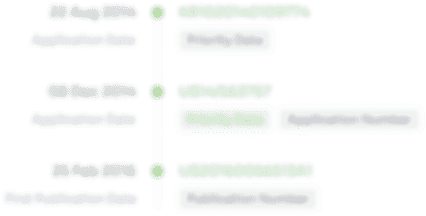
- R&D Engineer
- R&D Manager
- IP Professional
- Industry Leading Data Capabilities
- Powerful AI technology
- Patent DNA Extraction
Browse by: Latest US Patents, China's latest patents, Technical Efficacy Thesaurus, Application Domain, Technology Topic, Popular Technical Reports.
© 2024 PatSnap. All rights reserved.Legal|Privacy policy|Modern Slavery Act Transparency Statement|Sitemap|About US| Contact US: help@patsnap.com