Meanwhile, speaker clustering method for deep representation learning and speaker category estimation is optimized
A clustering method and speaker technology, which is applied in the field of speaker clustering and voiceprint recognition, can solve problems such as unfriendly clustering algorithms and inability to obtain clustering results
- Summary
- Abstract
- Description
- Claims
- Application Information
AI Technical Summary
Problems solved by technology
Method used
Image
Examples
Embodiment
[0061] Such as figure 1 As shown, this embodiment discloses a speaker clustering method that simultaneously optimizes deep representation learning and speaker category estimation, including the following steps:
[0062] The first step: preprocessing and extracting I-vector features, the steps are:
[0063] Read in the speech samples to be clustered, and pre-emphasize through the first-order high-pass filter, the filter coefficient a is 0.98, and the transfer function of the first-order high-pass filter is:
[0064] H(z)=1-az -1
[0065] Use the Hamming window for framing, the length of each frame is 25ms, and the frame shift is 10ms;
[0066] Perform Fourier transform on the framed signal xt(n) to obtain the frequency domain signal:
[0067]
[0068] Perform Mel filtering on the frequency domain signal, where the Mel filter bank contains M triangular filters, the center frequency of each filter is denoted as f(m), and the frequency response of the mth triangular filter...
PUM
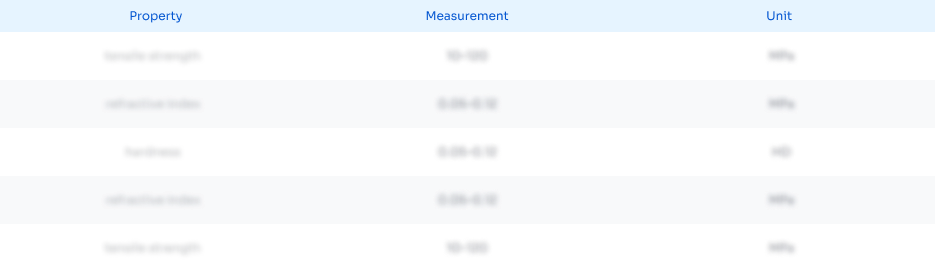
Abstract
Description
Claims
Application Information
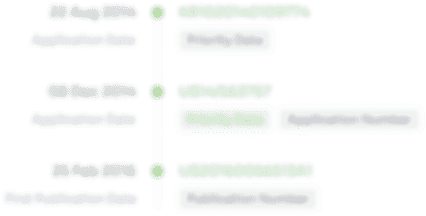
- Generate Ideas
- Intellectual Property
- Life Sciences
- Materials
- Tech Scout
- Unparalleled Data Quality
- Higher Quality Content
- 60% Fewer Hallucinations
Browse by: Latest US Patents, China's latest patents, Technical Efficacy Thesaurus, Application Domain, Technology Topic, Popular Technical Reports.
© 2025 PatSnap. All rights reserved.Legal|Privacy policy|Modern Slavery Act Transparency Statement|Sitemap|About US| Contact US: help@patsnap.com