Power distribution network fault classification method and system based on deep learning, and medium
A distribution network fault and deep learning technology, applied in information technology support systems, photovoltaic power generation, character and pattern recognition, etc., can solve problems such as poor protection sensitivity and complicated threshold value setting process, and achieve high classification accuracy and fast Reliable identification and classification, and the effect of reducing the false action rate
- Summary
- Abstract
- Description
- Claims
- Application Information
AI Technical Summary
Problems solved by technology
Method used
Image
Examples
Embodiment 1
[0064] Embodiment one, as figure 1 As shown, a distribution network fault classification method based on deep learning includes the following steps:
[0065] S1: Obtain multiple original fault waveform data sets of the DC distribution network;
[0066] S2: Process each original fault waveform data group separately to obtain target sample data corresponding to each original fault waveform data group;
[0067] S3: Make all the target sample data into a data set, divide the data set into a training set and a test set, construct a deep learning network model, use the training set to train the deep learning network model, and obtain the original fault classification model;
[0068] S4: Using the test set to perform parameter tuning on the original fault classification model to obtain an optimized fault classification model;
[0069] S5: Obtain the real-time fault waveform data group of the DC distribution network, and process the real-time fault waveform data group according to ...
Embodiment 2
[0141] Embodiment two, such as Figure 8 As shown, a distribution network fault classification system based on deep learning, including data acquisition module, data processing module, model acquisition module, parameter optimization module and fault classification module;
[0142] The data acquisition module is used to acquire a plurality of original fault waveform data groups of the DC distribution network; it is also used to obtain real-time fault waveform data groups of the DC distribution network;
[0143] The data processing module is used to process each original fault waveform data group separately to obtain target sample data corresponding to each original fault waveform data group; it is also used to process the real-time fault waveform data group, Obtaining the fault data to be tested corresponding to the real-time fault waveform data group;
[0144] The model acquisition module is used to make all target sample data into a data set, and divide the data set into a ...
Embodiment 3
[0166] Embodiment 3. Based on Embodiment 1 and Embodiment 2, this embodiment also discloses a distribution network fault classification system based on deep learning, which includes a processor, a memory, and is stored in the memory and can run on the A computer program on a processor, when said computer program runs, it realizes as figure 1 The specific steps from S1 to S5 are shown.
[0167] Through the computer program stored in the memory and run on the processor, the fault classification of the DC distribution network of the present invention is realized, and the faults in the distribution network are quickly and reliably identified and classified by using the strong classification advantages of deep learning. The efficiency is high, and the classification accuracy is high, which is beneficial to reduce the misoperation rate of the protection action of the DC distribution network according to the fault.
[0168] This embodiment also provides a computer storage medium, wh...
PUM
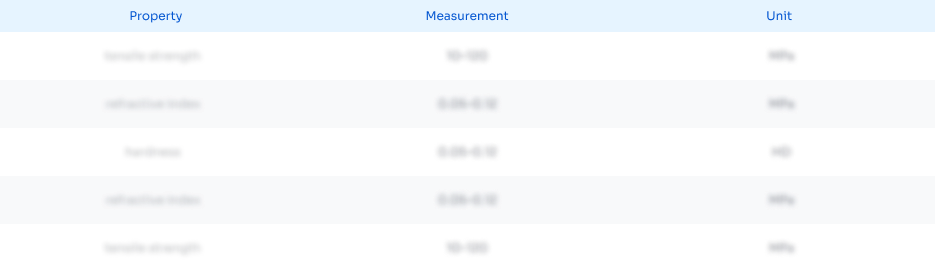
Abstract
Description
Claims
Application Information
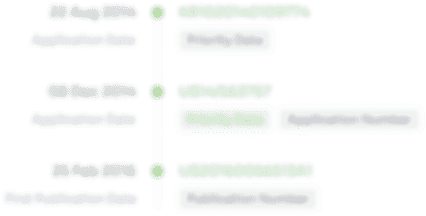
- R&D Engineer
- R&D Manager
- IP Professional
- Industry Leading Data Capabilities
- Powerful AI technology
- Patent DNA Extraction
Browse by: Latest US Patents, China's latest patents, Technical Efficacy Thesaurus, Application Domain, Technology Topic, Popular Technical Reports.
© 2024 PatSnap. All rights reserved.Legal|Privacy policy|Modern Slavery Act Transparency Statement|Sitemap|About US| Contact US: help@patsnap.com