Wind power medium-short term prediction method and system based on improved neural network
A neural network, short-term forecasting technology, applied in neural learning methods, biological neural network models, forecasting, etc., can solve problems such as wind power volatility
- Summary
- Abstract
- Description
- Claims
- Application Information
AI Technical Summary
Problems solved by technology
Method used
Image
Examples
specific Embodiment 1
[0118] The historical wind power of the entire wind farm and the above characteristic data are collected and organized as training data, and the current data feature vector and actual wind power are collected in real time as subsequent historical data; among them, the characteristic data include: wind farm The real-time characteristic data and the actual wind power corresponding to the real-time characteristic data are collected once at the first preset time interval, such as the first preset If the duration is set to 30 minutes, the data will be collected every 30 minutes.
[0119] Further, as figure 2 As shown, using "unsupervised learning" to cluster wind turbines belongs to a kind of neural network. Each subset is a group, and each group will have a strong association with some potential factors.
[0120] For data set D={x 1 ,x 2 ,...,x m}, containing m unlabeled fan samples, each sample x i =(x i1 ,x i2 ,...,x in ) is an n-dimensional eigenvector, that is, the e...
specific Embodiment 2
[0164] Step 1: Collect the historical wind power and predicted wind power of a wind farm and the characteristic data values affecting wind power: wind direction, air temperature, air pressure, humidity, wind speed, wind turbine height, wind turbine blade diameter, and wind turbine geographical location.
[0165] Step 2: According to the neural network clustering K-means algorithm, iteratively group the wind turbines in the wind farm.
[0166] Step 3: Carry out external and internal measurement of the fan grouping results. If there are external indicators, Jaccard index, FM index, and Rand index can be used. The larger the three index values, the better the clustering effect; the internal index DB index can also be used The Dunn index is used to measure, the smaller the DB, the larger the Dunn, the better the clustering and grouping effect.
[0167] Step 4: According to the historical characteristic data value and the real wind power, use the BP algorithm to establish the win...
PUM
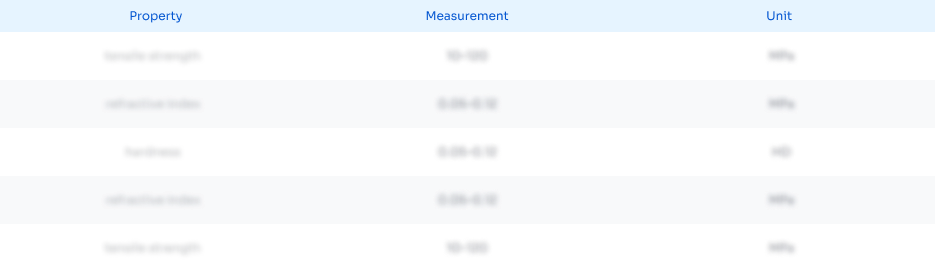
Abstract
Description
Claims
Application Information
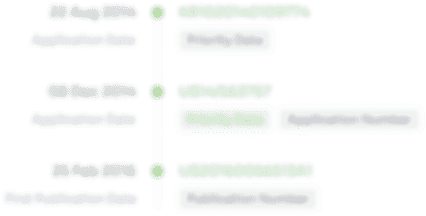
- R&D Engineer
- R&D Manager
- IP Professional
- Industry Leading Data Capabilities
- Powerful AI technology
- Patent DNA Extraction
Browse by: Latest US Patents, China's latest patents, Technical Efficacy Thesaurus, Application Domain, Technology Topic, Popular Technical Reports.
© 2024 PatSnap. All rights reserved.Legal|Privacy policy|Modern Slavery Act Transparency Statement|Sitemap|About US| Contact US: help@patsnap.com