Scene recognition and classification method based on Tiny-Darknet
A technology of scene recognition and classification method, which is applied in the field of scene recognition and classification based on Tiny-Darknet, can solve the problems of inability to meet real-time performance or low-end monitoring equipment, poor universality, and large memory footprint, so as to achieve a small memory footprint. , The effect of strong universality and high real-time performance
- Summary
- Abstract
- Description
- Claims
- Application Information
AI Technical Summary
Problems solved by technology
Method used
Image
Examples
Embodiment Construction
[0029] The present invention will be described in detail below with reference to the accompanying drawings and examples.
[0030] like figure 1 As shown, a Tiny-Darknet-based scene recognition classification method includes the following steps:
[0031] S1. Build a training sample set for the classification model;
[0032] S2. Build a deep learning framework based on the Tiny-darknet network;
[0033] S3. Configure the training parameters and train the classification model;
[0034] S4. Obtaining equipment monitoring image information;
[0035] S5. Classify scene images;
[0036] S6. Judging scene changes;
[0037] S7. Complete the scene recognition parameter configuration.
[0038] When constructing the training sample set of the classification model in step S1, a large number of images of different scenes are obtained from various scenes of actual application of the monitoring equipment and public data sets on the Internet, and these images are rotated at multiple angl...
PUM
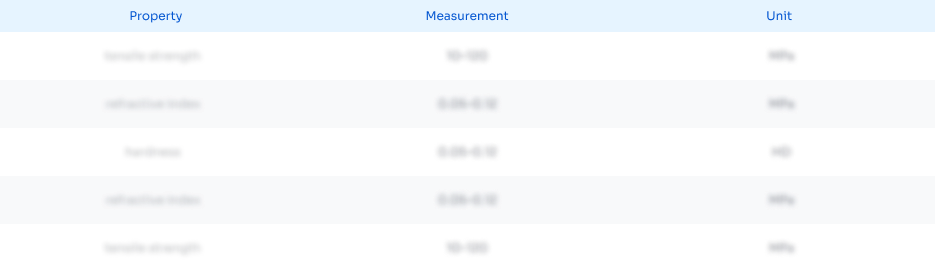
Abstract
Description
Claims
Application Information
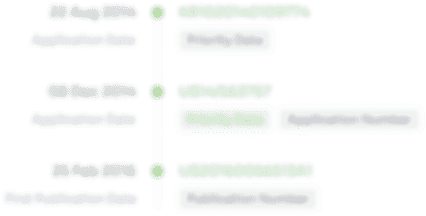
- R&D Engineer
- R&D Manager
- IP Professional
- Industry Leading Data Capabilities
- Powerful AI technology
- Patent DNA Extraction
Browse by: Latest US Patents, China's latest patents, Technical Efficacy Thesaurus, Application Domain, Technology Topic, Popular Technical Reports.
© 2024 PatSnap. All rights reserved.Legal|Privacy policy|Modern Slavery Act Transparency Statement|Sitemap|About US| Contact US: help@patsnap.com